How to Transform Your Business Decisions With Predictive Modeling
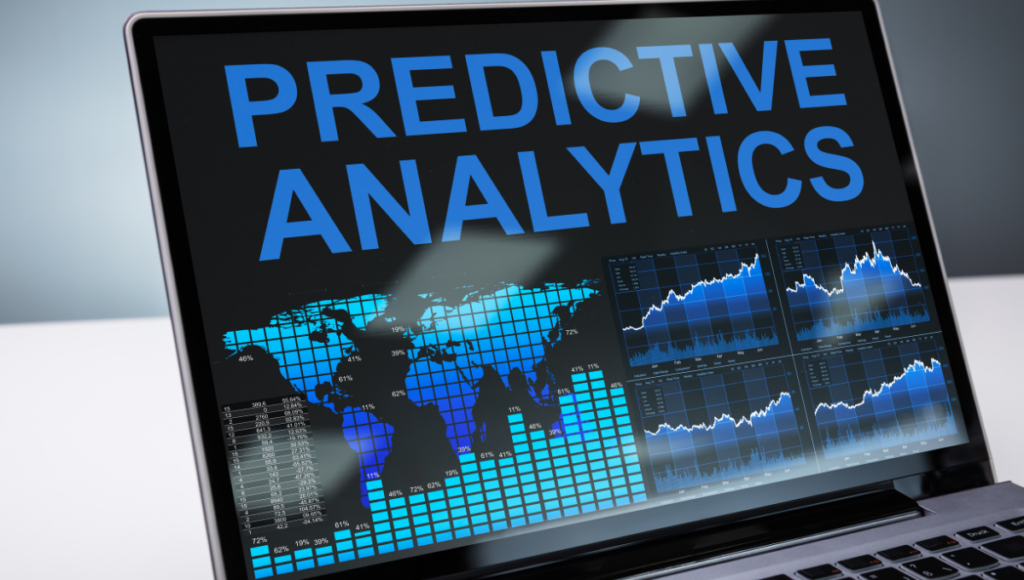
An organization’s decision-making is crucial for its progress, especially in today’s data-driven economy. This is probably why firms are always looking for ways to bolster their insights while making business decisions. For instance, data analysis has emerged as a reliable way to inform key decisions, decluttering information without bias; 53% of corporate leaders increased their investment in advanced analytics, which covers everything from predictive modeling to machine learning, according to a 2022 survey by McKinsey & Company. Predictive modeling is a powerful tool, enabling firms to stay ahead of the competition using historical data and statistical algorithms. So let’s learn how it can leverage data to see into the future and revolutionize the business strategy.
What is Predictive Modeling?
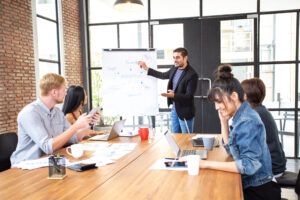
Predictive modeling is a technique that uses mathematical processes to predict future events or outcomes. It comes under predictive analytics, a strand of data analysis, that uses current and historical data to forecast behavior and trends. For instance, firms can estimate the probability of someone clicking a link or buying a product using predictive modeling, among other things.
There are two types of models—unsupervised and supervised. The former uses traditional statistics such as decision trees, logical regression, and time-series analysis to classify the data directly. The latter deploys newer machine learning techniques, such as neural networks, to recognize patterns buried in labeled data. Let’s take a closer look at what this is useful for:
ALSO READ: A Guide to Business Analysis: 7 Skills to Boost Your Analytics Career
1. Identify Patterns
The technique analyzes data to sniff out hidden patterns and trends. For example, it can reveal factors that lead to high sales with the help of past sales records.
2. Build Models
A firm can create a mathematical model with the help of patterns. It acts like a prediction machine, crunching new data to dictate future business decisions.
3. Ascertain Outcomes
Predictive modeling is also used to forecast the future. The technique can also predict outcomes apart from facilitating what-if analysis.
ALSO READ: 10 Most Popular Business Analysis Techniques Used by Professionals
How is it Different From Traditional Analytics?
1. Prediction
Predictive modeling forecasts future events or trends based on historical data patterns. In contrast, traditional analytics uses descriptive analysis to understand past and current trends.
2. Purpose
Predictive modeling is often used for strategic planning because it provides insights into future outcomes. Traditional analytics is used for reporting, performance monitoring, and identifying areas for improvement with the help of historical data.
3. Analysis
Predictive modeling chiefly uses more complex techniques to identify patterns and relationships in data to make predictions. However, traditional analytics uses elementary techniques such as summary statistics and visualization to illustrate data trends.
4. Data Requirement
A firm needs large and diverse data sets to train models effectively under predictive modeling. Traditional analytics, on the other hand, requires small data sets or can focus on specific metrics without the need for predictions.
5. Outcome Orientation
A business uses predictive modeling to speculate specific outcomes or events, such as customer behavior, market trends, or equipment failure. Traditional analytics reveals patterns and relationships within data without delivering predictions.
ALSO READ: 15 Key Business Analytics Tools You Can Master to Maximize Data Potential
How Can Predictive Modeling Benefit Businesses in Their Decision-Making Processes?
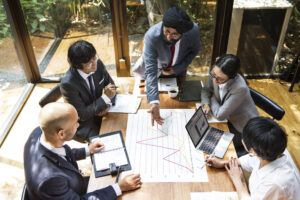
Businesses are often inundated with data. This is why they use business intelligence to harness data. Let’s take a look at the benefits of using predictive modeling for organizations:
1. Informs Decision-Making
A firm generates data-driven insights with predictive modeling that inform everything from resource allocation to marketing strategies. In other words, the insights are akin to having a crystal ball for your business.
2. Augments Efficiency
The technique helps to optimize business operations. For instance, companies can maintain adequate inventory levels, reduce stockouts, and allocate optimal resources. Moreover, they can anticipate equipment failures and order preventive maintenance to avoid costly disruptions.
3. Minimizes Risk
A business can discern potential problems before they affect the business. For example, manufacturing firms can gauge supply chain disruptions and stock up on raw materials. Similarly, insurance companies can predict high-risk claims and adjust premiums accordingly.
4. Boosts Marketing
A business can understand customers on a deeper level by analyzing past purchases and online behavior. It can build customer profiles based on their preferences and tailor their marketing campaigns subsequently.
5. Offers Competitive Advantage
Companies need to differentiate themselves to gain a significant edge in the market. They can use predictive modeling to study market trends and develop innovative products and services that cater to changing needs.
ALSO WATCH: Online Course Preview | Business Analytics: From Data to Decisions— Imperial College Business School
What are the Key Steps in Implementing Predictive Modeling in a Business Setting?
1. Define the Scope
A business must outline the objectives of its business intelligence process. It must be clear on the specific business problems that predictive modeling aims to solve and determine the areas where insights can provide value.
2. Prepare Data
The next step is gathering relevant data from various sources to feed the model. The data should be cleaned and analyzed to uncover patterns and potential outliers. It ensures accuracy and consistency in the model.
3. Select Features
It is crucial to convert raw data into necessary features for the model to deliver reliable predictions. Businesses can use feature engineering to combine and create new features that enhance the model’s performance.
4. Develop Models
Then, choose appropriate predictive modeling techniques based on the problem’s nature and the available data. It should train models with historical data, tune parameters, and evaluate their performance using appropriate metrics.
5. Validate Performance
The validation of predictive models using separate datasets is important to assess their accuracy. Evaluate the models against predefined performance metrics and criteria to determine their effectiveness in predicting outcomes reliably.
6. Deploy Models
Integrate predictive models into existing systems, processes, or decision-making workflows. The stakeholders should have access to the insights generated by the models in an actionable format.
7. Monitor Output
There must be a framework in place to monitor the model’s performance by tracking accuracy and impact on business outcomes in real-world scenarios. It should retrain models periodically to adapt to changing data patterns or business dynamics.
Transform Your Career With Emeritus
The number of businesses relying on data-driven insights is only set to increase in the coming days. To stay in step with a transformational landscape, consider one of Emeritus’ business analytics courses. These courses are taught by experts with practical knowledge of the actual challenges facing businesses today. Enroll for one of these courses today and stay ahead of the curve.
Write to us at content@emeritus.org