Mastering the Extract Transform Load Process: Best Practices for Effective Data Warehousing
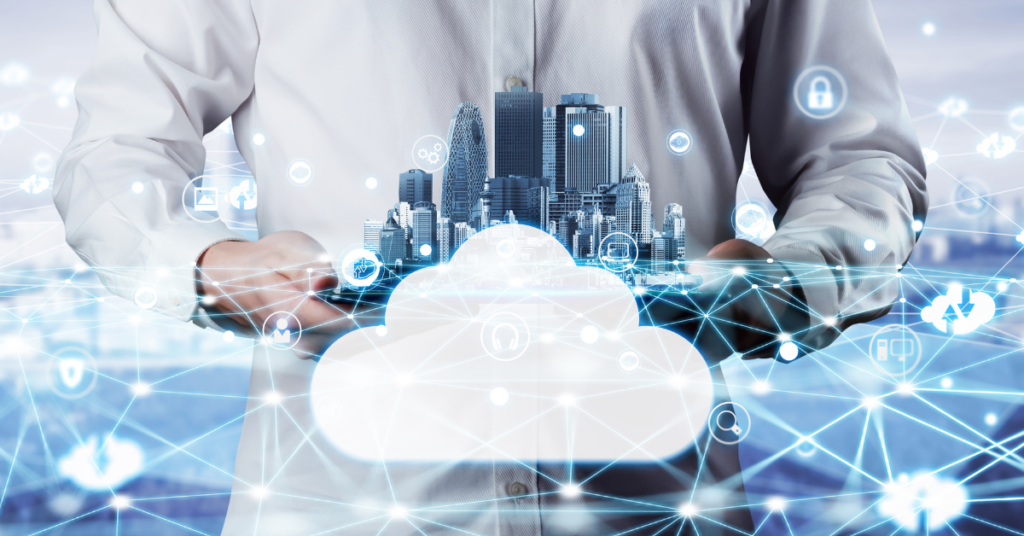
- What is the Purpose of the Extract Transform Load Process in Data Warehousing?
- What are the Key Components of the ETL Process?
- How Does the ETL Process Ensure Data Integrity in Data Warehousing?
- What are Some Common Challenges in Implementing the ETL Process?
- How Can Organizations Optimize Their ETL Processes for Better Performance?
The Extract Transform Load process, also called ETL, a key player in data warehousing, is witnessing a remarkable market surge. Valued at $3.1 billion in 2021, the global ETL software market is on track to hit $10.3 billion by 2030, marking a growth rate of 14.3% from 2022 to 2030. This blog takes a deep dive into the ETL process, unpacking its essential components, its challenges, and strategies for effective optimization. Tailored for data engineers, professionals, and managers, it offers insights into navigating the complexities of this evolving field.
What is the Purpose of the Extract Transform Load Process in Data Warehousing?
1. Understanding the Core of Data Warehousing
At its core, the Extract Transform Load process is pivotal in data warehousing. Essentially, it acts as a critical mechanism. First, it gathers data from many sources and refines it for accuracy and consistency. Finally, it adeptly stores it in a data warehouse.
2. Facilitating Efficient Data Storage and Accessibility
ETL does more than just store data. Indeed, it facilitates efficient data storage, a key aspect in today’s data-driven world. Moreover, it ensures that the data is not just stored but also readily available. This availability is crucial for analysis and decision-making, pivotal in driving business strategies.
3. Bridging Data Collection and Business Intelligence
The extract transform load process connects the dots between data collection and business intelligence. By doing so, it stores data and transforms it into a valuable asset. Moreover, this transformation is essential for businesses to make informed decisions, underscoring the significance of the extract transform load process in data warehousing.
ALSO READ: A Guide to Business Analysis: 7 Skills to Boost Your Analytics Career
What are the Key Components of the ETL Process?
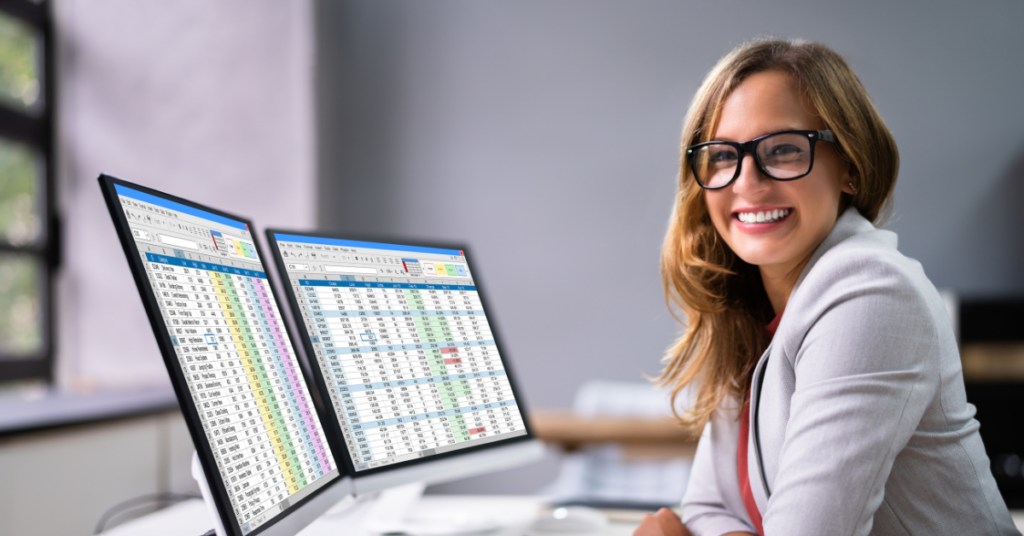
1. Data Extraction
The extract transform load process begins with extraction. In this initial phase, the process involves gathering data from diverse sources. These sources vary widely, from traditional databases to modern cloud-based systems.
2. Ensuring Data Quality Through Transformation
Next comes the transformation stage. Here, the extracted data undergoes a thorough cleansing process. This does not just mean cleaning the data; it also gets normalized and aggregated. This step is vital for ensuring data quality and relevance, making the data not only cleaner but also more meaningful and useful.
3. Completing the Process With Data Loading
Finally, the extract transform load process culminates with the loading phase. Here, the transformed data is carefully transferred to the data warehouse, making it readily accessible and primed for analysis. Hence, it marks the completion of a cycle that began with data migration from various sources to a centralized, structured repository.
How Does the ETL Process Ensure Data Integrity in Data Warehousing?
1. Ensuring Accuracy Through Data Validation
Maintaining data integrity is a top priority in data warehousing, and the extract transform load process plays a pivotal role in ensuring this. This is down to the fact that during the transformation phase, data validation is a key step where each piece of data is meticulously checked for accuracy.
2. Cleansing for Consistency and Reliability
The extract transform load process involves thorough data cleansing. In essence, this process involves removing or correcting any inaccuracies or inconsistencies in the raw data, transforming it into a valuable, reliable asset. Thus, cleansing ensures that the data loaded into the warehouse is not just accurate but also consistent and dependable.
3. Data Modeling and Consolidation
Data modeling and data consolidation, two fundamental functions of the ETL process, contribute significantly to data integrity. In essence, data modeling structures the data effectively, while consolidation ensures that all relevant data is brought together coherently.
ALSO READ: 15 Key Business Analytics Tools You Can Master to Maximize Data Potential
What are Some Common Challenges in Implementing the ETL Process?
1. Navigating Data Quality Hurdles
One of the most common challenges in the extract transform load process is ensuring data quality. As data is extracted from diverse sources, it often comes with inconsistencies and errors. This is akin to sifting through a mixed bag—finding and correcting inaccuracies is crucial but can be daunting.
2. Overcoming Integration Complexities
Integration complexities often pose significant hurdles. Since the extract transform load process involves merging data from various systems, the integration phase can be complex. Furthermore, this complexity is not just technical; it also involves aligning different data formats and structures, a task similar to piecing together a multi-shaped puzzle.
3. Maintaining the ETL Pipeline Efficiency
Maintaining the efficiency of the ETL pipeline is another challenge. As data volumes grow and business needs evolve, the ETL pipeline must adapt and scale efficiently. This requirement is like tuning an engine for optimal performance—it demands continuous attention and fine-tuning.
4. Addressing the Expanding Data Integration Market
The surge in the data integration software market, which grew by 9% in 2022 to hit $4.6 billion, underscores a rising demand for extract transform load processes. However, this expansion introduces complexities, primarily due to the integration of diverse and voluminous data sources. Therefore, it necessitates advanced ETL solutions that can efficiently process and harmonize various data formats and structures.
5. Ensuring Successful Data Migration
Successful data migration also poses a critical challenge because transferring data from legacy systems to modern platforms requires careful planning and execution. Therefore, data migration is not just a simple transfer. Rather, it is also about ensuring data integrity and compatibility with new systems.
ALSO READ: Learn How to Earn a Lucrative Salary as a Business Analyst
How Can Organizations Optimize Their ETL Processes for Better Performance?
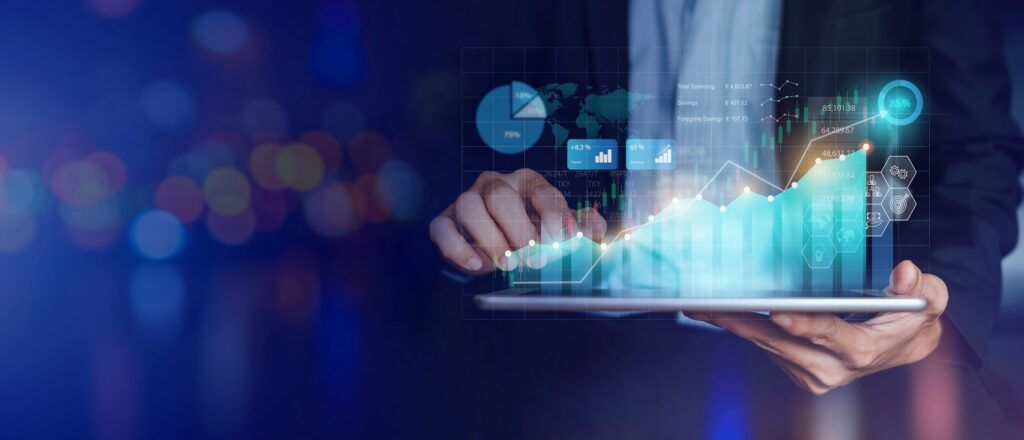
1. Implementing Comprehensive Logging
Effective logging is crucial in the ETL process. Essentially, it involves meticulously recording each step, which aids in monitoring the process flow and identifying any issues promptly. Moreover, logging helps maintain a clear record for future reference, ensuring that every data movement and transformation is traceable and transparent.
2. Rigorous Auditing for Data Integrity
Auditing in ETL is essential for optimizing their ETL processes. In essence, it involves a thorough review of data handling and processing. This step ensures that the data adheres to set standards and complies with regulatory requirements, thereby safeguarding the integrity of the data throughout the ETL pipeline.
3. Establishing Robust Data Lineage
Data lineage is about understanding the data’s journey through the ETL process. Establishing robust data lineage means having a clear view of the data’s origin, what transformations it undergoes, and where it moves. This clarity is crucial for data validation and helps maintain data quality and accuracy.
4. Setting up Effective Alerts
Alerts are critical for proactive ETL management. They notify teams of any anomalies or failures in the process, allowing for immediate action. Thus, setting up effective alerts ensures that any issues are addressed swiftly, minimizing the impact on the overall ETL process and data migration.
5. Utilizing Parallel Processing
Parallel processing can significantly enhance ETL performance. By processing multiple data streams simultaneously, it speeds up the data transformation and loading phases. This approach is particularly effective in handling large volumes of data, ensuring efficiency and scalability. Using automation to perform this task can benefit organizations looking to optimize their ETL processes.
6. Leveraging Cache Data
Caching data can optimize ETL performance by reducing the time taken to access frequently used data. In essence, it acts as a temporary storage for quick retrieval, speeding up the process, especially during complex data modeling and data consolidation tasks.
ALSO READ: What is Descriptive Analytics and Why a Data-Driven World Needs it
The extract transform load process is critical in data warehousing, driving data quality, efficiency, and business intelligence. As the global data warehousing market, valued at $21.18 billion in 2019 and projected to reach $51.18 billion by 2028, continues to grow, the importance of ETL becomes ever more significant. Enrolling in Emeritus’ business analytics courses can be a valuable step forward for professionals looking to deepen their understanding and skills in this area.
Write to us at content@emeritus.org