Why Diagnostic Analytics is the Hero You Need
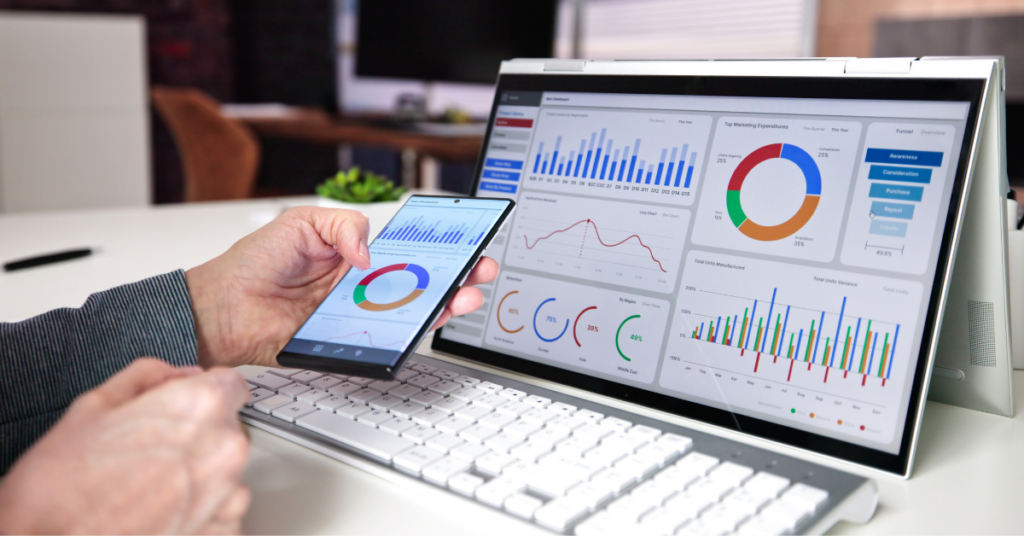
Imagine you notice your grocery bills have been creeping up lately. Descriptive analytics simply tells you how much you’ve been spending, but despite shuffling things, you are not able to keep things within budget. This is where the power of diagnostic analysis lies. It is like having your very own consulting detective on the case. Investigating the data at hand, it might reveal you’re buying more expensive organic produce, or that there’s been a recent price hike on your favorite brand of cereal. By understanding the “why” behind the rising costs, you can make informed decisions, like trying store brand alternatives or planning your meals more strategically. That is the power of diagnostic analysis—it gives you the insights you need to solve problems and make smarter choices.
For example, if sales are slumping, diagnostic analysis might reveal a correlation between the decline and a recent marketing campaign that missed the target audience. Or, if a website is experiencing high bounce rates, it could pinpoint technical glitches or confusing navigation that is frustrating users. By uncovering these root causes, businesses can make informed decisions to improve marketing strategies, enhance user experience, and ultimately achieve their goals. In short, diagnostic analysis is the key to unlocking the hidden stories within your data, empowering you to solve problems and make data-driven decisions for success.
Understanding Diagnostic Analytics
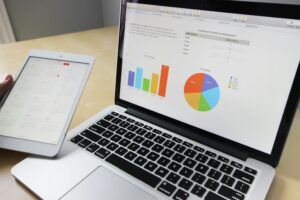
Diagnostic analytics essentially goes beyond simply describing what is happening (descriptive analytics) or predicting what might happen (predictive analytics). In contrast, it delves into the why, helping you identify the factors influencing trends and outcomes. Think of it as peeling back the layers of an onion to reach the core cause.
Here is where diagnostic analytics differs from its analytical kin:
- Descriptive analytics tells you what happened (e.g., sales figures for the last quarter)
- Predictive analytics tries to forecast what might happen (e.g., predicting future sales trends)
- Prescriptive analytics suggests actions to optimize outcomes (e.g., recommending marketing strategies to boost sales)
However, diagnostic analytics focuses on the reason behind those sales figures or trends. It answers questions such as, Why did sales drop in Q2? or What factors are influencing this sales prediction?
The practice of diagnostic analytics incorporates several key activities:
1. Data Mining
This involves the use of sophisticated data analysis tools to discover patterns and relationships in large datasets that might not be apparent through simpler analysis techniques.
2. Drill Down
This technique allows analysts to view data at increasing levels of detail to pinpoint where anomalies are coming from, breaking down aggregated data to granular details.
3. Data Discovery
Often associated with exploratory data analysis, this component involves looking through data to find unknown correlations and hidden patterns without a specific hypothesis in mind.
Each component of diagnostic analytics serves to deepen the understanding of business operations and outcomes, facilitating a more nuanced and informed approach to data-driven decision-making. This detailed insight is what allows organizations to not just react to past events but to proactively manage future challenges and opportunities, tailoring strategies that are informed by comprehensive data analysis.
ALSO READ: 5 Best Practices and Tips for Designing Data-Intensive Applications
How Diagnostic Analytics Works: The Process
Imagine investigating a sudden drop in website traffic. Here’s a step-by-step look at the process involved in diagnostic analysis:
Identifying the Anomaly
You notice a dip in traffic using website analytics (descriptive analytics).
Data Collection and Processing
You gather data on user behavior, page views, and potential website issues.
Analysis With Statistical Techniques
You use tools such as correlation analysis to identify possible causes for the traffic drop.
Interpreting the Results
You discover a correlation between the traffic drop and a recent website redesign.
Leveraging Visual Representation
Charts and graphs help you clearly see the relationship between the redesign and traffic decline.
Turning to Human Expertise for Insights
While data tells the story, your experience helps you determine if the redesign actually caused the drop or if there is another factor at play.
ALSO READ: Anomaly Detection: Why it’s Important for Data Scientists
Best Industry Examples of Diagnostic Analytics
Diagnostic analytics is more than just a theoretical framework. It is a reliable guide that helps businesses across various industries make informed and insightful data-driven decision-making. Here is how some leading businesses are leveraging its power:
1. Health Care
Imagine a hospital struggling with high patient readmission rates. This can be a frustrating situation for both patients and health-care providers. Fortunately, diagnostic analytics can be a powerful tool in this scenario. By analyzing patient data, including medical history, medication adherence, and social determinants of health, hospitals can gain valuable insights. This analysis allows them to identify patients at risk for readmission. Armed with this knowledge, they can then implement targeted interventions, such as medication reminders or post-discharge support programs. Ultimately, this leads to improved patient outcomes and reduced medical costs.
2. Retail
Diagnostic analysis can also become your retail bloodhound, sniffing out the root cause of sales dip or other issues. In a 2023 article by Forbes, highlighted the myriad ways diagnostic analysis can help make business decisions with the greatest impact. Suppose you noticed stagnant sales of a particular shoe line you launched. The data detectives can dig deeper and see if there is a mismatch between the advertised demographic and the actual customer buying the shoes. This analysis can help understand the “why” behind slow sales, thereby helping you adjust your marketing campaigns to reach the right audience, potentially boosting sales and preventing future inventory pileups. Furthermore, diagnostic analysis empowers retailers to make data-driven decisions on everything from product selection to store layout, ultimately leading to happier customers and a healthier bottom line.
3. Finance
In 2023, Capital One, a major US bank, fell victim to a data breach that exposed the personal information of millions of customers. Now imagine if Capital One used diagnostic analysis, it could analyze patterns in the accessed data, pinpointing specific types of information targeted by the hackers. This data detective work could have revealed a focus on Social Security numbers or dates of birth, suggesting the attackers aimed for identity theft. Additionally, diagnostic analysis could have identified the source of the breach, like a vulnerability in a specific system or a compromised employee login.
By uncovering these root causes, Capital One could not only notify affected customers but also take targeted action to prevent future attacks. This might involve patching the exploited system vulnerability, implementing stricter access controls, or even collaborating with law enforcement to track down the perpetrators. The Capital One case thus demonstrates the critical role diagnostic analysis plays in financial security.
4. Manufacturing
In addition to streamlining current processes, diagnostic analytics can analyze sensor data from machinery to predict equipment failures before they occur. This enables proactive maintenance, preventing costly downtime and production delays. Furthermore, by analyzing product quality data, manufacturers can pinpoint the root cause of defects. This root cause analysis leads to improved production processes and higher-quality products, ultimately strengthening a manufacturer’s competitive edge.
5. E-commerce
Enhancing customer experience goes beyond website navigation patterns. It is important to understand the entire customer journey. Diagnostic analytics can be a powerful tool in this endeavor. It goes deeper than just looking at the number of clicks. By analyzing customer search queries, abandoned shopping carts, and product reviews, online retailers can gain valuable insights into customer needs and pain points. This deeper understanding allows them to personalize product recommendations, a strategy that can significantly improve customer satisfaction. Additionally, diagnostic analytics can be used to improve search functionalities to match user intent better, ensuring customers can easily find what they are looking for. Finally, by analyzing product reviews, retailers can address customer concerns efficiently, demonstrating responsiveness and building trust. Ultimately, these combined efforts translate to a smoother customer journey and increased customer loyalty, creating a positive feedback loop that benefits both the retailer and the customer.
ALSO READ: 10 Ways in Which Data Science Can Make Healthcare Better
Implementing Diagnostic Analytics in Your Business
Diagnostic analytics is undeniably a powerful tool, but wielding it effectively requires careful planning. Before diving headfirst into diagnostic analytics, consider these factors to be your key to success:
A. Data Quality is Key
Imagine building a house on a foundation of sand. The structure would be weak and unstable, destined for failure. Similarly, diagnostic analytics is only as good as the data it feeds on. Dirty or incomplete data will lead to flawed insights and, ultimately, poor decision-making. Therefore, ensuring your data is accurate, complete, and consistent is crucial. Invest in data-cleaning processes to scrub away errors and inconsistencies. Furthermore, establish data governance policies to maintain data integrity over time. By following these steps, you will lay a solid foundation for your diagnostic analytics efforts, ensuring they deliver valuable and actionable insights.
B. Build a Strong Foundation With Tech Infrastructure
Diagnostic analytics requires a robust technology infrastructure to handle the complexities of data analysis. This infrastructure could include data warehouses to store vast amounts of data, acting as the digital vault for your information. In addition, Business Intelligence (BI) tools provide the necessary functionality for data analysis and visualization, allowing you to transform raw data into insights you can understand. Finally, depending on the scalability of the data, cloud computing resources can offer the scalability needed to process and analyze massive datasets efficiently. By investing in a robust technology infrastructure, therefore, you equip yourself with the tools necessary to unlock the full potential of diagnostic analytics.
C. Skilled Personnel
Having skilled data analysts and data scientists on your team is crucial. These individuals possess the expertise to interpret the insights gleaned from diagnostic analytics, acting as translators who can unlock the meaning hidden within the data. Furthermore, they can translate these insights into actionable business strategies, transforming data-driven discoveries into practical steps that propel your business forward. Moreover, by investing in skilled personnel, you have the necessary expertise to utilize diagnostic analytics effectively and maximize its value for the organization.
Here is a step-by-step guide to get you started:
1. Define the Problem or Opportunity
First and foremost, define the problem. Is it high customer churn, declining sales in a specific product category, or operational inefficiencies? Clearly define the area where diagnostic analytics can provide the most value.
2. Data Collection and Preparation
Secondly, gather relevant data from various sources, such as Customer Relationship Management (CRM) systems, sales data, website analytics tools, and social media platforms. Clean and organize the data to ensure it is ready for analysis.
3. Choose the Right Tools
Remember, you need the right tool for the job. The specific tools you use will depend on your data type, budget, and technical expertise. Some common options:
- SQL is a powerful query language for extracting and manipulating data from relational databases and the foundation for many data analysis workflows.
- Python is a versatile programming language with a vast ecosystem of libraries specifically designed for data analysis, machine learning, and data visualization. Options like Pandas, NumPy, and Matplotlib empower you to wrangle, analyze, and visualize your data.
- R is a statistical programming language ideal for complex statistical analysis and data modeling. It is particularly popular in academic and research settings.
- Tableau and Power BI are user-friendly BI tools that offer drag-and-drop functionality for data visualization and exploration. They allow you to create interactive dashboards and reports, making data insights accessible to a wider audience within your organization.
ALSO READ: What is Data Science? Why is This Career Path in Demand? Find Out Now!
4. Analyze and Draw Insights
Use your chosen tools to uncover patterns and trends within your data. Statistical analysis techniques like correlation analysis, regression analysis, and hypothesis testing can help identify potential root causes.
5. Take Action
Finally, based on your findings, implement changes to address the root cause of the problem. This could involve anything from launching targeted marketing campaigns to optimizing product features to streamlining internal processes.
Remember, diagnostic analytics is an ongoing process. As you gather more data and refine your techniques, you will gain a deeper understanding of your business and unlock its full potential.
ALSO READ: Why is it Important to Learn Python in Data Science?
Diagnostic analytics is a powerful tool that empowers you to see beyond the surface of your business data. It is almost like revealing the hidden truths that drive success (or signal trouble). Therefore, by understanding the why behind trends and metrics, you can make data-driven decisions that optimize operations, improve customer experiences, and propel your business forward.
The future of business strategy is, therefore, undeniably tied to the power of analytics. As the saying goes, “You can’t improve what you don’t measure.” Diagnostic analytics takes measurement a step further—it delves into the why, giving you the power to not just measure but truly understand. Data is becoming increasingly relevant, and it won’t be any exaggeration to say that learning more about diagnostic analytics, along with other data concepts, will illuminate your path to success. The future of your business might just depend on it. So, explore Emeritus’ online data science courses and get ahead of the game.
Write to us at content@emeritus.org