Anomaly Detection: Why It’s Important for Data Scientists
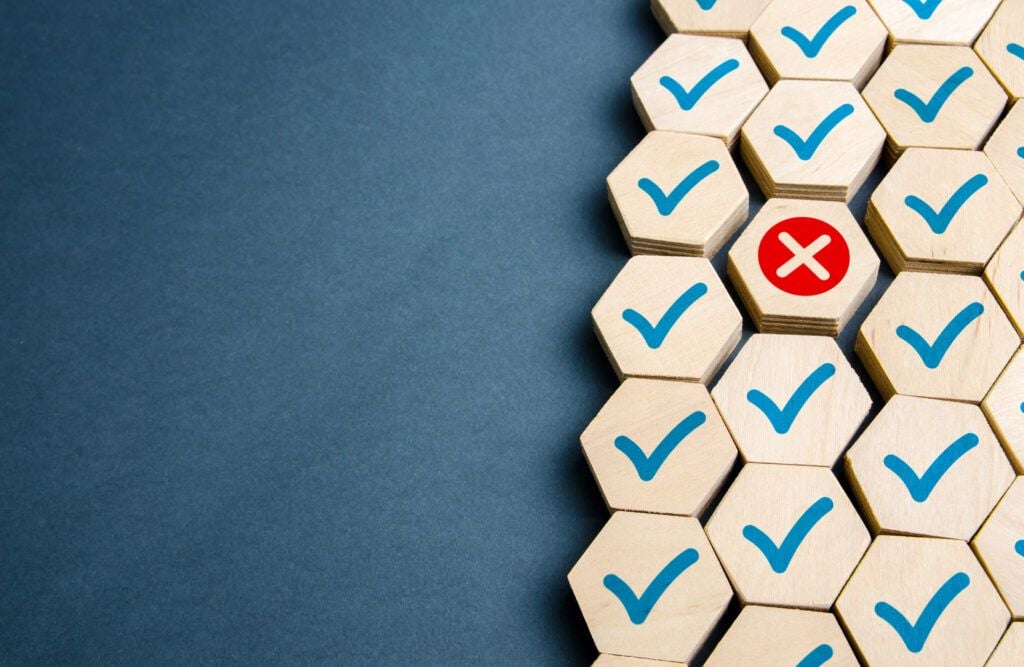
In today’s increasingly interconnected and digital-centric society, data is the ultimate guiding force behind many of our decisions. From multinational corporations using analytics to shape their business strategies to individuals tracking their daily steps on fitness apps, data undeniably constitutes our world. Amidst this vast sea of information, the importance of tools and techniques for screening, understanding, and deriving meaning from this data cannot be overstated. Chief among these is anomaly detection, a technique that is steadily gaining prominence.
For data scientists, this isn’t merely another tool in the shed but a critical instrument to ensure data accuracy and integrity. Moreover, this escalating importance doesn’t seem to be just a passing trend either. Reflecting its growing significance, the global anomaly detection market is on an upward trajectory, with projections estimating its worth to be a staggering $5.11 billion in the current year. Given such impressive figures and increasing reliance on it, one naturally can’t help but wonder: what exactly is anomaly detection and why is it rapidly becoming the talk of the town in the data realm?
In this blog, you will learn:
- What is Anomaly Detection and Why is It Important for Data Scientists?
- How Does Anomaly Detection Benefit Data Analysis and Decision-Making Processes?
- What are the Common Challenges and Techniques Involved in Anomaly Detection?
- How Can Data Scientists Effectively Apply Anomaly Detection in Their Work?
- Looking to Enhance Data Science Skills? Start With an Emeritus Data Science Course
What is Anomaly Detection and Why is It Important for Data Scientists?
Anomaly detection is a method to pinpoint unusual patterns that do not align with expected behaviors. These irregularities are often called outliers or anomalies. Essentially, think of it as a system raising a red flag when something doesn’t seem right. Let’s look at why it is important in the context of the impact it can have in our daily lives, and for data scientists.
1. The Real-World Implications of Anomalies
Interestingly, anomalies are not mere statistical aberrations; they often have tangible real-world implications. For instance, consider scenarios like a sudden spike in traffic to a website, a drop in sales, or a surge in energy consumption. Or, to make it even more relatable, imagine your credit card company flagging an unusually hefty transaction made in a foreign country when you haven’t traveled abroad. Such anomalies might very well signal potential fraud, indicating a compromised card. Similarly, anomaly detection can help identify network intrusions, highlighting unauthorized access in the critical realms of cybersecurity. It can show system failures in industrial sectors, predicting a machinery breakdown before it occurs.
2. Crucial for Data Scientists
Understanding and mastering anomaly detection, first and foremost, has multifaceted benefits. On one hand, it ensures the purity and accuracy of data by identifying and rectifying outliers. This aspect is crucial, especially when building predictive models, where data accuracy directly impacts the effectiveness of predictions. On the other hand, anomaly detection aids in identifying underlying issues or trends that might otherwise go unnoticed. This proactive identification can subsequently give businesses the foresight to prevent potential problems or capitalize on emerging opportunities.
ALSO READ: 5 Best Practices and Tips for Designing Data-Intensive Applications
3. Accuracy in Data Analysis
Data analysis, at its core, revolves around the unending quest for truth and understanding. Within this quest, accuracy plays the lead role, shaping the narrative of the data story being told. Precisely, this is the place where anomaly detection helps. Acting as a meticulous auditor, anomaly detection diligently filters vast data sets, ensuring no outlier goes unnoticed. For data scientists, this implies that their analytical models and algorithms are built upon a foundation of clean, robust data, free from the misleading influence of outliers. After all, the most accurate insights are undeniably derived from the purest data.
ALSO READ: Key Differences Between Data Analyst and Data Scientist: Which Role is Better?
4. Data-Driven Decisions
In modern business, “data-driven” isn’t just a buzzword; it is a mantra. Every strategic move and decision invariably hinges on the insights extracted from data. But what happens when this data is skewed? The ripple effects of a single incorrect decision based on flawed data can be undeniably monumental, leading to financial losses or missed opportunities. In this context, anomaly detection plays a pivotal role, ensuring businesses navigate the complex market landscape accurately and precisely. It guarantees that every step taken is grounded in reliable, accurate information.
5. Anomaly Detection on the Rise
Businesses today have understood the significance of data and its accuracy in making informed decisions. The trajectory is unmistakably clear, with 87.8% of businesses amplifying their data investments in 2022. Moreover, at the beginning of this year, an anticipated 93.9% were set to further increase these investments in 2023. Amidst this booming focus on data, anomaly detection will likely play an even more pivotal role. As companies pour resources into extracting insights from data, ensuring the integrity of that data becomes critical. Anomaly detection, with its vigilant eye on outliers, will undeniably be central to this mission.
ALSO READ: A Deep Dive Into Data Lakes: 7 Best Practices for Data Management
What are the Common Challenges and Techniques Involved in Anomaly Detection?
Anomaly detection is much like solving a puzzle. There are different strategies to spot the piece that doesn’t fit. Let’s delve into the commonly used techniques below.
1. Statistical Techniques
Imagine, for instance, that you’re accustomed to seeing a range of numbers, like scores on a test from 70 to 100. Suddenly, there is a score of 200. That’s how statistical techniques work. They understand what’s “normal” based on basic math rules. If something breaks these rules, it stands out. However, there’s a caveat. When data becomes more varied and multilayered, these techniques can occasionally struggle to pinpoint anomalies with precision.
2. Clustering-Based Methods
Consider the analogy of organizing a room. The toys are in one corner, books on a shelf, and clothes in a wardrobe. Now, if a toy is found among the clothes, it’s clearly out of place. Clustering methods work similarly by grouping similar data points. Anything that doesn’t fit within these groups is perceived as unusual. Yet, it can be complex because sometimes it is challenging to decide which group a particular item belongs to.
3. Machine Learning-Based Methods
Anomaly detection is similar to adjusting the sensitivity on a modern touchscreen device. It requires precise calibration to respond to subtle changes in input accurately. Thus, machine-learning-based methods enable computers to learn from past data, aiding them in making predictions about new data. For anomaly detection, it is fundamentally about teaching the computer what’s “normal” and then allowing it to flag what seems odd. It’s a dynamic approach, adapting to new patterns over time. Nevertheless, it requires using ample past data to train, which makes the whole process of anomaly detection more intricate.
ALSO READ: 5 Ethical Aspects for Data Science Professionals to Consider
How Can Data Scientists Effectively Apply Anomaly Detection in Their Work?
1. Understanding the Data is the Key
First and foremost, understand your data inside out. The foundation of effective anomaly detection is a comprehensive grasp of the data set one is working with. It is necessary to know the nature of the data, its patterns, history, and, importantly, potential pitfalls.
2. Select the Right Tool
Choosing the right tool for the job is pivotal. With a myriad of techniques available, it’s essential to align the method with the nature of the data and the specific anomalies one is hunting for.
3. Iterative Process is Crucial
Remember, anomaly detection is inherently iterative. It is not a one-and-done affair. As new data pours in and patterns evolve, it is crucial to revisit, reevaluate, and fine-tune the detection mechanisms. This is an ongoing journey, demanding continual vigilance and adaptability.
4. Establishing Accurate Thresholds
Having clear thresholds is an essential component in ensuring accurate anomaly detection. By creating well-defined thresholds, data scientists can better identify what constitutes an “anomaly” in their data.
ALSO READ: 10 Best Online Data Science Courses Hand-Picked for You
Looking to Enhance Data Science Skills? Start With an Emeritus Data Science Course
As the field of data science continues to evolve, professionals must equip themselves with tools and techniques that enable them to derive meaningful insights from data while maintaining its integrity. So, to deepen the understanding and harness the power of data analytics, explore Emeritus’ data science courses and take the next step in your professional journey today!
Write to us at content@emeritus.org