A Deep Dive Into Data Lakes: 7 Best Practices for Data Management
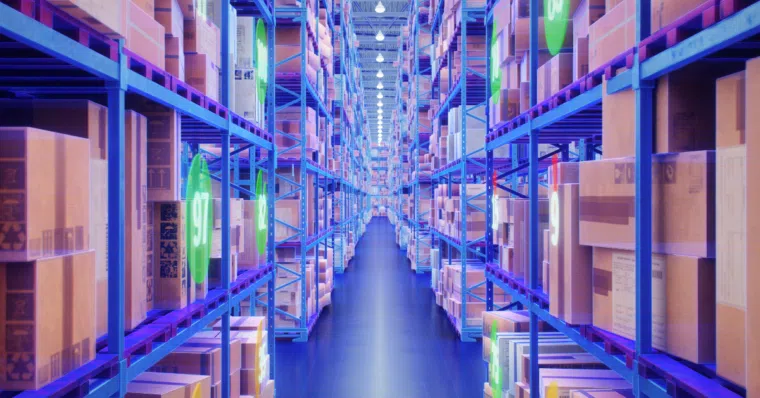
- What are Data Lakes, and How Do They Differ From Traditional Data Warehouses?
- What are the Key Challenges in Managing Data Lakes Effectively?
- What are the Best Practices for Designing a Scalable Data Lake Architecture?
- How Can Data Governance be Implemented in a Data Lake Environment?
- What Tools and Technologies Can be Used for Data Integration and Data Processing?
- How Can Emeritus Courses Help You Understand the Concept and Architecture of Data Lakes?
Given the wave of digitization across industries, data plays a crucial role in shaping businesses. It is the backbone of decision-making processes and a driving force behind innovation. In this ocean of data, a new concept called data lakes is making quite a splash. According to Mordor Intelligence, the market is set to witness substantial expansion, with projections indicating an increase from $13.74 billion in 2023 to $37.76 billion by 2028, reflecting a CAGR of 22.40% during the five-year forecast period. Numbers aside, let’s dive into understanding what data lakes are, and how they can be managed effectively.
ALSO READ: The Importance of Data Analytics: How Does it Benefit a Business?
What are Data Lakes, and How Do They Differ From Traditional Data Warehouses?
Data lakes are vast storage repositories that hold a massive amount of raw data in its native format until needed. Unlike traditional data warehouses that store structured data and require a defined schema before data ingestion, data lakes hold structured, semi-structured, and unstructured data. Furthermore, this allows businesses to capture a broader range of data types and sources, providing a more comprehensive view of business operations and customer behavior.
What are the Key Challenges in Managing Data Lakes Effectively?
Maintaining Data Quality
The presence of poor quality data, or ‘dirty data’, can lead to inaccurate analysis, consequently resulting in misinformed business decisions. Hence, ensuring high-quality data is crucial for any data lake environment.
Effective Data Governance
Additionally, implementing effective data governance within a data lake can be complex. The sheer volume, velocity, and variety of data flowing into the lake necessitate a robust governance strategy. Moreover, the absence of such a strategy can lead to data integrity, security, and usability issues, hindering effective data management.
Data Security and Privacy
Next, let’s talk about data security and privacy. Given the massive amounts of sensitive data stored in such databases, protecting it from unauthorized access and ensuring compliance with various data protection regulations becomes a major challenge. Hence, stringent security measures are non-negotiable.
Managing Data Storage and Retrieval
Another critical issue is managing data storage and retrieval. With a vast amount of data stored in a data lake, ensuring efficient data storage and swift retrieval can be tricky. Moreover, this directly impacts the speed of data analysis and insights generation.
Understanding Data Context
Lastly, understanding the context of the raw data stored in a data lake can be daunting. Without the right context, there’s a risk of misinterpretation of data, which could lead to erroneous conclusions and decisions.
ALSO READ: The Power of Data Analysis and How to Unlock Business Insights for Success
What are the Best Practices for Designing a Scalable Data Lake Architecture?
1. Adopting a Tiered Storage Strategy
The first step involves adopting a tiered storage strategy. This approach allows businesses to manage and store data based on its access frequency and importance. Therefore, it optimizes costs and improves the efficiency of data retrieval.
2. Implementing Metadata Management
This involves cataloging data with tags and descriptions, making it easily searchable and interpretable. By managing metadata effectively, businesses can enhance the user experience, making finding and understanding the data stored in the lake easier.
3. Incorporating Data Partitioning and Indexing
Incorporating data partitioning and indexing can significantly enhance data retrieval speed. In addition, businesses can improve their query performance by enabling the system to quickly locate and access the required data subset, thereby saving valuable time.
4. Designing for Scalability
Businesses can ensure that their data lake handles future data growth without performance degradation by designing the data lake, keeping scalability in mind. Additionally, this helps maintain data processing efficiency even as the data volume grows.
5. Ensuring Data Security
Data security is a crucial aspect of any data lake architecture. Therefore, implementing a robust security framework that includes encryption, access controls, and regular audits can protect the data lake from unauthorized access and potential data breaches.
6. Incorporating Data Governance
Effective data governance practices, such as data quality checks, data lifecycle management, and regulatory compliance, ensure that the data in the lake remains reliable and usable.
7. Choosing the Right Tools and Technologies
Lastly, choosing the right tools and technologies for data ingestion, storage, processing, and analysis can significantly enhance the performance and usability of the data lake. This undoubtedly helps businesses streamline their data management processes using the right technology stack and extract more valuable insights from their data lake.
How Can Data Governance be Implemented in a Data Lake Environment?
1. Develop a Data Catalog
Firstly, develop a data catalog: This acts as a central repository for all data assets, aiding users in gauging what data is available, its origin, and how it is interconnected.
2. Implement Access Controls
Next, implement robust access controls to uphold data security. By clearly defining who has permission to access specific data, you can prevent unauthorized data access and ensure adherence to data protection regulations.
3. Establish Data Quality Rules and Validation Processes
Moreover, you need to maintain data integrity in the lake. Frequent data quality checks can help spot and rectify data quality issues, thereby ensuring the reliability of your data.
4. Regularly Audit Data Usage
Lastly, conduct regular data usage audits to guarantee compliance with various regulations and standards. These audits can help pinpoint potential security risks and non-compliance issues, allowing you to address them promptly.
What Tools and Technologies Can be Used for Data Integration and Data Processing?
1. ETL Tools
Extract, Transform, and Load (ETL) tools should be a ‘must-have’ in your data integration tools list. These aid in extracting data from various sources, transforming it into a suitable format, and loading it into the data lake.
2. Big Data Processing Frameworks
On the processing front, big data processing frameworks such as Hadoop and Spark are commonly utilized for processing vast volumes of data. These handle batch and real-time data processing, making them appropriate for various workloads.
3. Data Lake Management Tools
Next up are data lake management tools that provide functionalities like data cataloging, data governance, and security. They help streamline your data lake management and ensure it remains a valuable asset rather than becoming a data swamp.
4. Data Query and Analysis Tools
Tools such as SQL and NoSQL databases, data warehousing services, and business intelligence tools can come in handy for querying and analyzing data in the lake. They also help you extract valuable insights from data, enabling better data-driven decision-making.
5. Machine Learning and Artificial Intelligence Tools
Lastly, machine learning and artificial intelligence tools can be leveraged to uncover hidden patterns and insights in data. These tools can help you make more accurate predictions, enhance decision-making, and drive innovation.
ALSO READ: How SQL for Data Science Helps With Data Visualization
How Can Emeritus Courses Help You Understand the Concept and Architecture of Data Lakes?
To sum up, it holds immense potential for organizations aiming to harness the power of data. However, effective management is critical to prevent your data lake from becoming a swamp. The comprehensive courses provided by Emeritus on data lake architecture, data management, and analytics have a hands-on, practical approach. Furthermore, these courses can empower you to understand and leverage the potential of data lakes effectively. So, if you want to master the intricacies of data analytics, start your learning journey with Emeritus’ data science courses.
Write to us at content@emeritus.org