Deep Learning vs. Machine Learning: The Ultimate Guide for 2022
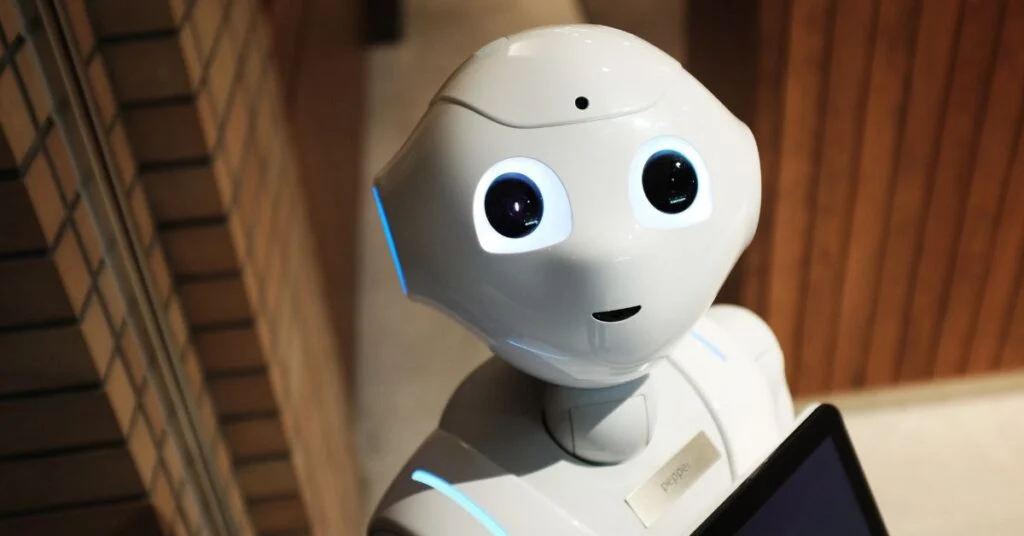
Always wondered how Amazon provides the best recommendations based on your previous purchases, or how Siri finds any information you are looking for in a matter of seconds? From self-driving cars to voice assistants, deep learning has made it all possible. Artificial neural networks and deep learning are changing how we function, inside and outside our homes. But first, let us find out the differences between deep learning vs machine learning.Â
How Does Deep Learning Work?
Deep learning is a subset of machine learning. Machine learning in turn is a subset of artificial intelligence.
Therefore, artificial intelligence > machine learning > deep learning.
Continuous data analysis and bucketing help companies visualize information better. Deep learning algorithms draw intelligent conclusions by analyzing data using a logical approach. This is similar to how humans would analyze and visualize data but at a considerably faster rate and with a lower error rate.Â
A multi-layered algorithm helps achieve logical structuring in deep learning. Moreover, such neural networks are the backbone of deep learning. Natural language processing (NLP), audio and speech recognition software, image recognition tools, and facial recognition too are all examples of deep learning.
How Does Machine Learning Work?
Machine learning algorithms are not new. Humans have been trying to program machines to think better and in a more logical way for decades now. However, the ability to apply complex algorithms to big data applications in a cost and time-efficient manner is a recent development. Also, companies that have harnessed this technology with skill and sophistication are already leading the race!Â
Machine learning experts have been focusing on helping computers learn and improve constantly based on previous experiences. Machine learning algorithms dig deep into data, identify patterns, and offer interesting insights, and trends; all with minimal human intervention. Also, the core requirement to automate computers using machine learning algorithms would be to ensure data-defined patterns, a defined set of rules, and clear objectives. Moreover, thousands of companies around the world have been able to successfully transform manual tasks like bookkeeping, or logging service requests into completely automated processes using machine learning.Â
Key Differences: Deep learning vs machine learningÂ
Deep Learning | Machine Learning |
Deep learning is a subset of machine learning. Additionally, machine learning has evolved to create deep learning. | Machine learning is a subset of artificial intelligence and a superset of deep learning. Artificial intelligence has evolved to create machine learning. |
Neural networks are used in deep learning for data representation. Also, big data is prevalent here and consists of millions of data points. | Structured data is used in machine learning to build algorithms. Also, machine learning has thousands of data points. |
The output can range from numbers to free-form elements like text or sound. | Output is always numerical values only |
Neural networks are used in deep learning to pass data through various layers for processing. Also, this is done to interpret better and find trends. | Model functions are built using automated algorithms. Also, this helps the machine predict better using available data. |
Deep learning solves complex machine learning issues and helps experts dive deeper. Moreover, this helps efficiently predict with minimum human involvement. | Machine learning is used to learn new things, identify trends, and stay ahead of the competition. Also, there is much more human intervention needed. |
Types of Machine Learning
Machine learning can be of four types namely supervised, semi-supervised, unsupervised, and reinforcement.Â
- Supervised As the name suggests, supervised learning is where the machine is taught by example.
- Semi-supervised – In this type of machine learning, using a healthy mix of labeled and unlabelled data, machines are taught to label unlabelled data and make information more comprehensible.Â
- Unsupervised – Machine learning algorithms are used primarily to identify patterns.Â
- Reinforcement – A set of actions, parameters, and end values are provided to machines.Â
Learn more about the four types of machine learning here.
Types of Deep Learning
A Deep Neural Network (DNN) is an Artificial Neural Network (ANN) with multiple layers between input and output. Moreover, the success of DNNs has led to lower error rates in speech and image recognition over the last decade.
There are three primary neural network types in deep learning:
1. Multi-Layer Perceptrons (MLP)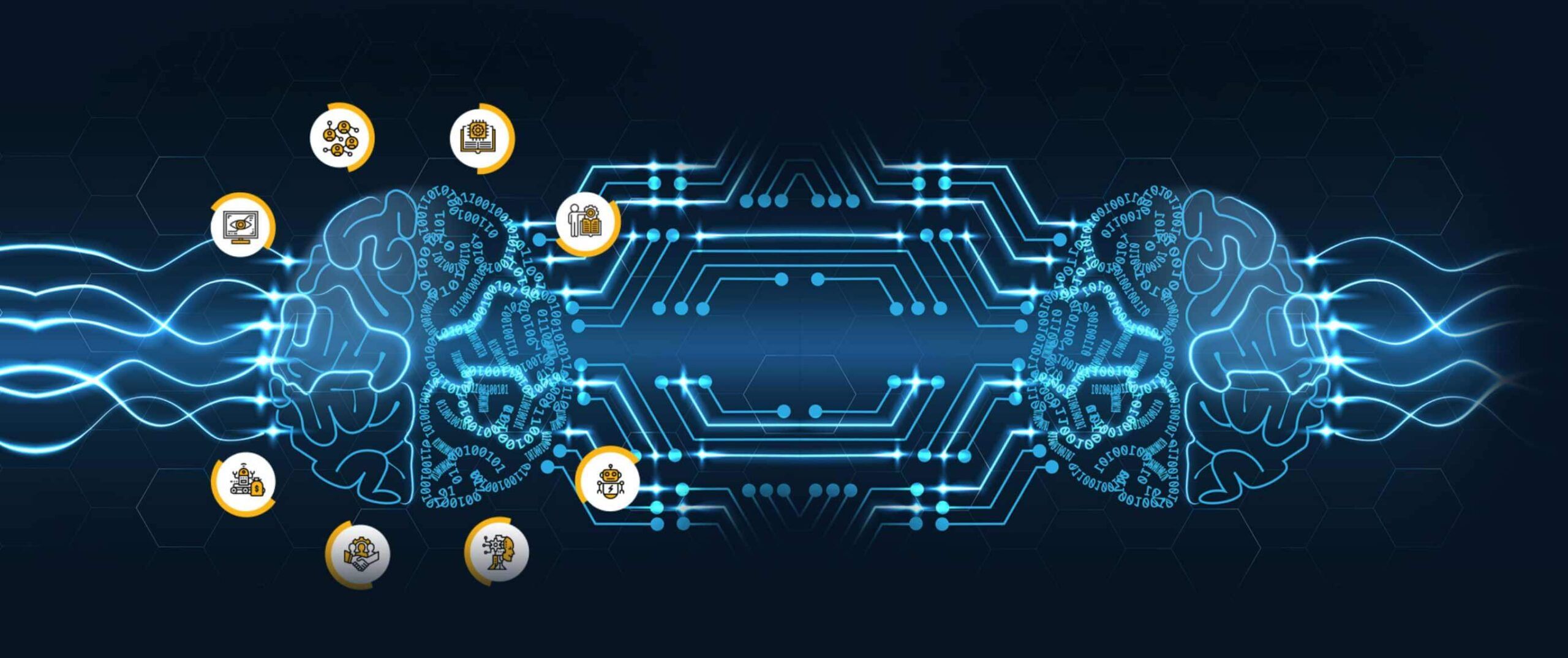
One of the most popular and basic feedforward artificial neural networks is MLP. It comprises a series of fully connected layers. Also, modern deep learning architectures use MLP to overcome the need for high computing power. Every new layer in an MLP is a set of non-linear functions. The weighted sum of all the fully connected outputs makes the next layer and so on.Â
2. Convolutional Neural Networks (CNN)
Unlike MLPs, CNNs are most commonly used in computer vision. Each layer is a set of non-linear functions but the weighted sums do not create the next layer. Instead, the weighted sums of different coordinates of output subsets that are spatially nearby from the previous layer lead to the next layer. Also, this allows the weights to be reused. Therefore, when a series of images or videos are shared, CNN learns to extract features from the input and classifies the output after due image recognition and object classification. Â
3. Recurrent Neural Networks (RNN)
This type of artificial neural network also uses sequential data feeding. However, RNN resolves the time-series issue of sequential input data. In the method, input includes previous samples. However, the connections between multiple nodes form a directed graph along a temporal sequence, unlike CNN or MLP. NLP is the most popular use case. Additionally, its superiority in processing data with varying input lengths makes it ideal for NLP. In such cases, artificial intelligence comprehends the input using modeling, embedding, or translation.
How to Improve Your Deep Learning vs Machine Learning Skills
With more and more companies moving toward digital transformation, it is no longer a good-to-have skillset. The world of artificial intelligence, machine learning, and deep learning is booming. Looking to advance your skills up the technology curve to solve more complex problems using artificial intelligence-driven solutions? Explore world-class online courses only on Emeritus. Also, all programs are in association with the world’s leading universities.Â
IDC forecasts the artificial intelligence market will break the $500 billion mark by 2024. This signals that the race for artificial intelligence-capable talent is on. Ready to explore? Click here to know more about all the artificial intelligence programs on Emeritus. As a learner, you’ll develop artificial intelligence problem-solving skills that are most necessary and applicable across a large range of information systems. This will help you and your organization stay competitive in this rapidly evolving landscape.Â
By Manasa Ramakrishnan
Write to us at content@emeritus.org