The Real Reason Behind AI Hallucinations and How to Combat Them
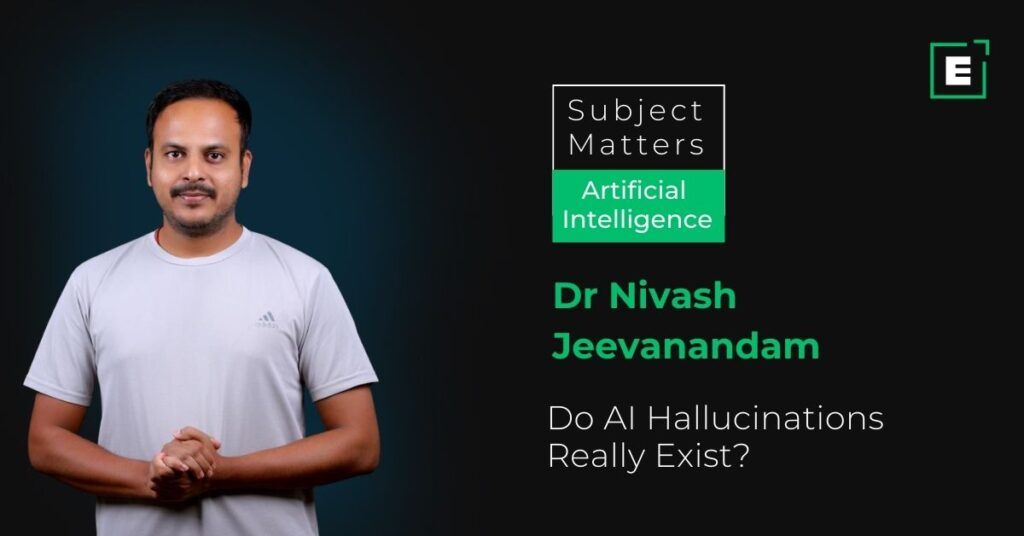
While AI hallucinations may sound like something out of a science fiction novel, they are becoming an increasingly relevant topic in AI research. As AI systems get more advanced and complex, they may display behaviors that imitate human perception, resulting in unexpected outputs or interpretations similar to the phenomenon of hallucinations in the human mind. To put it briefly, AI hallucinations refer to erroneous or deceptive outcomes that AI algorithms produce. These errors may arise due to several circumstances, such as inadequate training data, faulty assumptions made by the model, or biases in the data used for model training. In this article, we investigate the nature of AI hallucinations, their repercussions, and potential pathways for further research and mitigation.
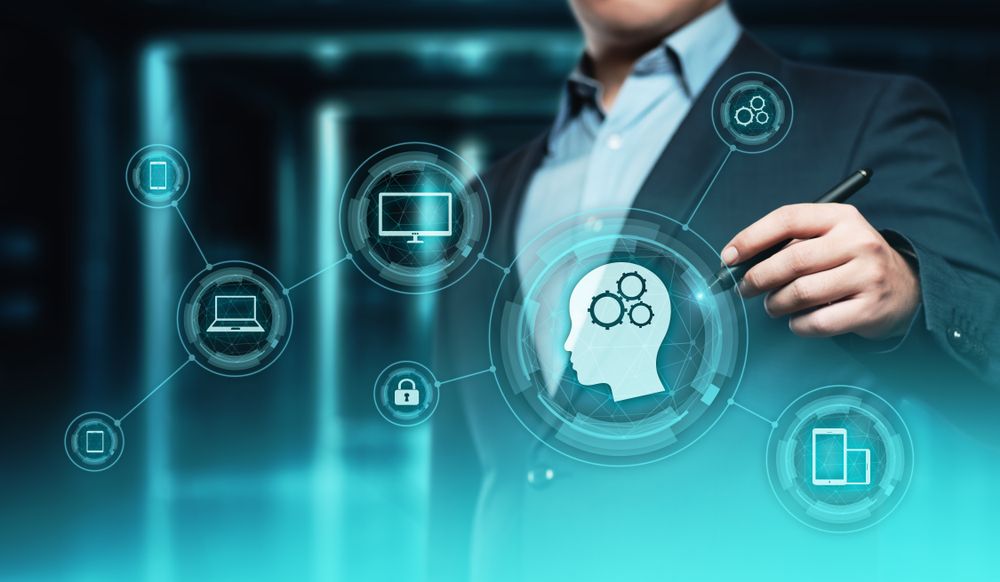
What are AI Hallucinations?
An AI hallucination commonly arises from adversarial instances. Simply put, this refers to diverse input data that confuses AI systems, causing them to misclassify and misinterpret the data, which further leads to inappropriate and hallucinatory output. The issue of AI hallucination poses a challenge as it undermines the user’s confidence in the AI system, thus detrimentally affecting decision-making. As a result, this potentially leads to various ethical and legal dilemmas. One possible method to address the issue of AI hallucinations is to enhance the training inputs by incorporating a wide range of diverse, accurate, and contextually relevant data sets. Another solution is gathering frequent user feedback and involving human reviewers to evaluate the AI systems’ outputs.
The Cause for AI Hallucinations
AI hallucinations occur when algorithms, data, and training methods interact to yield unexpected results. These hallucinations can take many forms, including visual aberrations in image recognition systems, illogical replies in natural language processing models, and incorrect predictions in decision-making algorithms.
A variety of circumstances can cause AI hallucinations. One major problem is the inherent restrictions and biases in the training data that is used to build AI algorithms. Such biases can result in distorted representations of the real world, thus causing AI systems to make inaccurate assumptions or linkages. Furthermore, the complexity of deep learning architectures can lead to unexpected emergent behavior, in which the model creates outputs that the researchers did not explicitly intend or predict.
Examples of AI Hallucinations
AI hallucinations can take a variety of forms. Some common examples are:
Incorrect Predictions
An AI model may forecast an event that is unlikely to occur. For example, an AI weather prediction model may predict that it will rain tomorrow despite the fact that no rain is anticipated.
False Positives
When using an AI model, it may incorrectly identify something as a threat. For example, an AI model employed to identify fraud may label a transaction as fraudulent even if it is not.
False Negatives
An AI model may fail to recognize something as a threat when it actually is. For instance, an AI model meant to diagnose cancer may fail to identify a malignant tumor.
Now, let’s investigate what are hallucinations in Large Language Models (LLMs).
A large language model is a trained machine learning model that generates text in response to the command you supply. The model’s training gave it some information gleaned from the training data provided. It is difficult to determine what knowledge a model remembers or does not. In fact, a model cannot know if the text it generates is correct.
In the context of LLMs, “hallucination” refers to a phenomenon in which the model generates language that is wrong, illogical, or untrue. Since LLMs are neither databases or search engines, they do not state where their responses are based. These models produce text as an extrapolation of the prompt you provide. The outcome of extrapolation is not always supported by training data, but it is the most linked with the prompt.
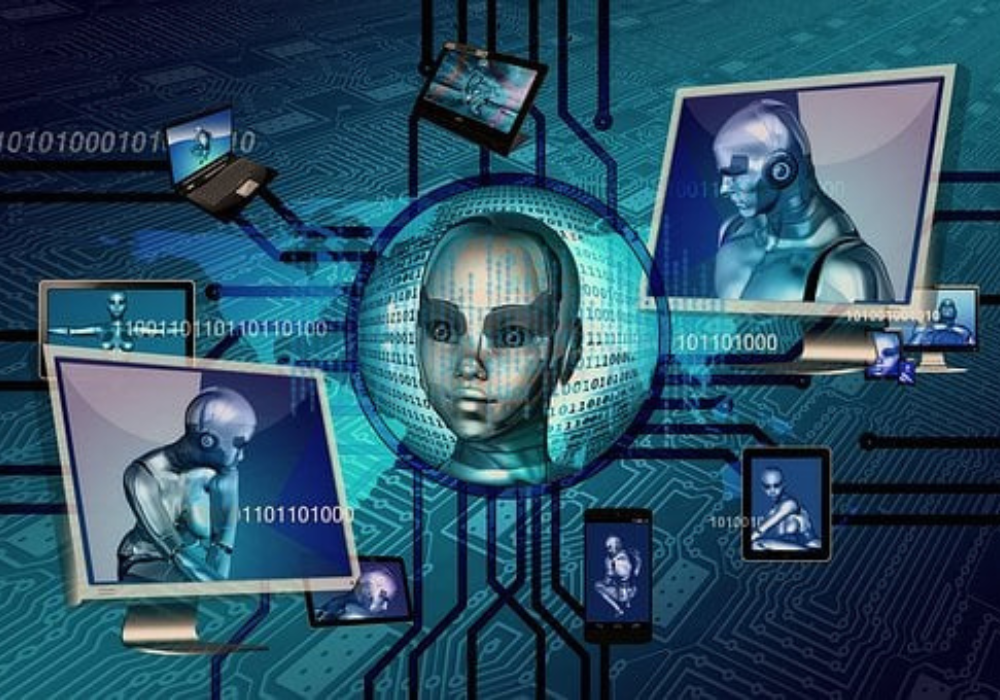
Types of LLM Hallucinations
The hallucinations in the context of LLMs are as follows:
- Input-conflicting hallucination occurs when LLMs generate content that differs from the source input provided by users
- Context-conflicting hallucination is when LLMs generate content that contradicts previously generated information by themselves
- Fact-conflicting hallucination is one in which LLMs create information that contradicts conventional world knowledge
AI Hallucination and its Impact on ChatGPT
This phenomenon poses a significant obstacle to the application of ChatGPT when it comes to scientific writing and analysis. However, it is possible to reduce its impact. Enhancing the training inputs for AI models can mitigate significant data drifts, temporal degradation, and AI hallucinations. This can be achieved by utilizing verified, accurate, and contextually relevant data sets instead of relying solely on a large volume of data. Continuous user feedback from credible sources on a nominal scale should also be provided. Nevertheless, retraining models and detecting early signs of deterioration might present difficulties, such as catastrophic forgetting and failure to converge.
These obstacles are particularly prominent in critical fields such as health care and scientific literature. Hence, it is crucial for further investigation to ascertain patterns of erroneous or omitted citations. Most importantly, although ChatGPT and other AI systems are trustworthy, they should not be the primary source for generating research proposals for medical or scientific journals. Individual writers must bear responsibility for any erroneous results from using ChatGPT in scientific writing.
Implications and Issues
AI hallucinations present essential issues in multiple disciplines. Erroneous AI system outputs in critical applications such as health care or autonomous vehicles could have potentially fatal repercussions. AI hallucinations also present ethical questions about accountability and transparency, as stakeholders must grasp or explain the reasons behind these unusual behaviors.
Mitigation Strategies
Managing AI hallucinations demands a multifaceted strategy. To begin with, improving the diversity and quality of training data can reduce biases. They can also increase the robustness of AI systems. Moreover, incorporating interpretability and explainability techniques into AI models can reveal insights into decision-making. This will allow for more accurate detection and correction of hallucinatory results. Ongoing research into adversarial training approaches and algorithmic fairness strives to improve the dependability and trustworthiness of AI systems.
AI hallucinations are a fascinating yet challenging frontier in AI research. Understanding the fundamental causes of these events, and their implications, is necessary. It will allow academics and practitioners to strive toward constructing more trustworthy, transparent, and ethical AI technology. Continued interdisciplinary collaboration and innovation are required to navigate this complicated landscape and realize AI’s full potential for society’s benefit.
NOTE: The views expressed in this article are those of the author and not of Emeritus.