What is Artificial Intelligence Optimization? All You Need to Know
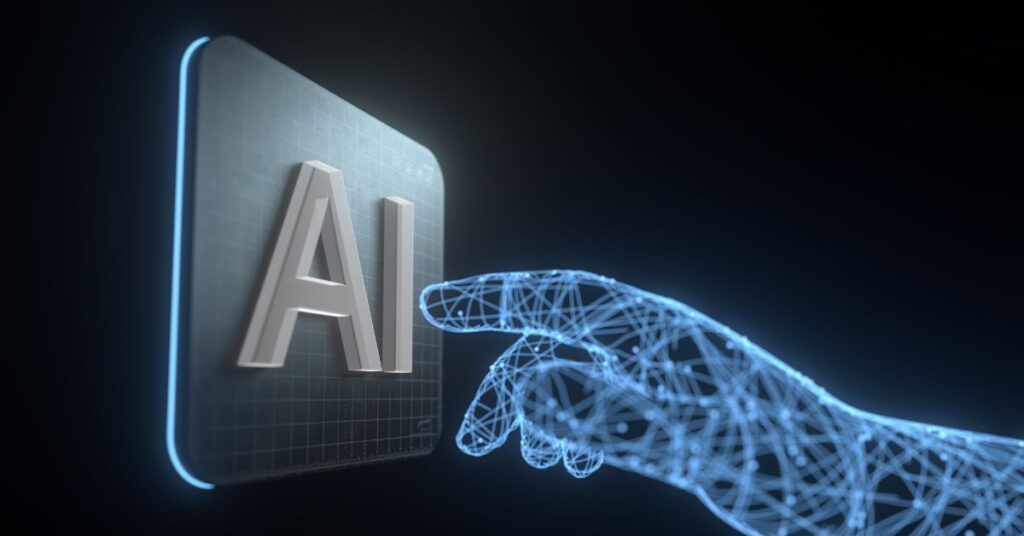
- What is Artificial Intelligence Optimization and Why is it Important?
- How Can AI Optimization Improve the Performance of Machine Learning Algorithms?
- What are the Key Factors to Consider When Optimizing AI Models?
- How Does AI Optimization Contribute to Better Decision-Making in Digital Marketing?
- What are the Latest Trends and Advancements in AI Optimization Techniques?
The rapidly evolving technological landscape identifies Artificial Intelligence (AI) as a transformative force, especially in India. According to a report published in The Economic Times, the growth rate of India’s AI market is 25–35% annually; accordingly, experts predict it will reach around $17 billion by 2027. This is certainly not hard to believe. AI has already proved its value by enhancing efficiency and improving decision-making processes. However, maximizing these benefits hinges on one critical factor: the optimization of AI models to ensure peak operational efficiency and effectiveness for all AI systems. This blog post actively investigates the intricacies of artificial intelligence optimization, its role in shaping the future of business organizations, its various techniques, and its latest trends.
What is Artificial Intelligence Optimization and Why is it Important?
1. What is Artificial Intelligence Optimization?
In the domain of AI development, artificial intelligence optimization plays a pivotal role: it necessitates fine-tuning AI models to enhance their efficiency. This undertaking further refines machine learning algorithms, which learn from data with unprecedented precision. Through AI optimization, developers strive to minimize computational demands and guarantee the peak performance of AI systems. The crucial fine-tuning process enables the creation of scalable, robust AI applications that can adeptly handle diverse challenges. This approach ultimately promotes the development of resource-efficient yet powerful AI solutions.
2. Why is it Important?
Primarily, artificial intelligence optimization accelerates information processing and decision-making in AI systems; thus, their speed and responsiveness to tasks increase substantially. Moreover, through optimization, AI developers gain the ability to address complex problems with heightened accuracy, a distinct advantage that propels technological advancements further. It critically facilitates the scalability of AI solutions, empowering them to efficiently dissect expansive data sets. Furthermore, these applications can be tailored with unprecedented precision via meticulous AI model tuning, meeting specific user requirements. In essence—optimization is integral not just for boosting system efficiency but also for maximizing versatility and adaptiveness in all aspects of artificial intelligence.Â
ALSO READ: Unleashing the Power of AI for Job Search: A Comprehensive Guide
How Can AI Optimization Improve the Performance of Machine Learning Algorithms?
1. Enhancing Operational Efficiency
Artificial intelligence optimization enhances operation efficiency through multiple mechanisms. Foremost among them is code optimization, which reduces memory and CPU usage. This strategic reduction in infrastructure costs precipitates several benefits; most notably, adjustments to a model’s design speed up result production times, thus facilitating faster decision-making.Â
2. Improving Model Effectiveness
Directly optimizing model effectiveness enhances the accuracy and reliability of AI outputs. For example, when we retrain models with higher-quality data, their decision accuracy rates significantly improve. Moreover, by addressing AI model tuning for effectiveness—a process that ensures adaptability to changes—we maintain not just relevance but also utility over time.Â
3. Addressing Model Drift
Machine learning algorithms face a substantial challenge in the form of model drift. To begin, environmental changes that engender inefficiencies can be mitigated through AI optimization strategies. Moreover, retraining models actively counter data drift and guarantee optimal inferences from the model are not affected. Maintaining the model’s efficiency and effectiveness over time requires essential, regular efforts in AI optimization; these safeguard against inevitable shifts within data environments and operational contexts.
4. Deployment Modification
Deploying models in a modified manner can immediately enhance performance. For instance, when we deploy a model on more robust hardware, its performance is temporarily augmented. This approach must supplement deeper optimization endeavors that tackle the fundamental causes of inefficiency.
5. Source Code Enhancements
Enhancing a model’s source code may yield substantial performance improvements: adopting more efficient libraries or frameworks can boost AI efficiency. This strategy, though demanding significant effort, proves worthwhile for specific models due to the potential of enhanced performance.
6. Regularization
Regularization tackles underfitting and overfitting by adjusting the model’s weight on different data types. This optimization enhances its data interpretation capabilities, guaranteeing accurate decisions based on real-world inputs.
ALSO READ: Responsible AI: Top 9 Aspects of Building Ethical AI Systems
What are the Key Factors to Consider When Optimizing AI Models?
Several factors enhance AI efficiency and AI performance. Some of them are presented below:Â
1. Data Quality and Relevance
Crucial to artificial intelligence optimization are the quality and relevance of training data. High-quality data ensures accurate pattern learning. Furthermore, the relevance of the data to the problem improves model effectiveness. Therefore, we understand that foundational selection in this process is actually all about data.
2. Algorithm Selection
The efficiency of AI hinges on selecting the appropriate algorithm: various machine learning algorithms cater to distinct problems, with some exhibiting superior computational effectiveness. Consequently, choosing the right algorithm is pivotal for achieving optimal performance in AI.
3. Model Complexity
Balancing the complexity of models is key to effective AI model tuning. Underfitting may occur with overly simplistic models, whereas overfitting can result from excessively complex ones. Thus, finding an optimal balance is absolutely essential.
4. Computational Resources
The vital to optimal artificial intelligence optimization is the precise utilization of computational resources. The speed of training and efficiency of models hinge on the availability of computational resources.
5. Regularization Techniques
L1 and L2 regularization techniques, crucial to AI optimization, actively encourage the development of simpler models. Thus, they play a vital role in preventing overfitting and ensuring generalization.
6. Hyperparameter Tuning
Methods such as grid search enable the discovery of optimal model parameters, a crucial step in tuning AI models for efficiency and accuracy. Hyperparameter tuning significantly amplifies performance. Therefore, it is an indispensable aspect of AI model enhancement.
7. Continuous Monitoring and Updating
Maintaining AI efficiency necessitates continuous monitoring and updating of the models. Regular retraining to address data drift guarantees sustained accuracy and reliability in the model performance.
ALSO READ: Will AI Replace SEO: What Can We Guess From 10,000 Years of Human History?
How Does AI Optimization Contribute to Better Decision-Making in Digital Marketing?
1. Streamlining Campaigns and Minimizing Errors
Artificial intelligence optimization automates operations in digital marketing, streamlines campaigns, and minimizes human errors. This AI-powered automation simulates the problem-solving and decision-making capabilities of humans, thus providing data-driven insights that enhance our understanding of customer needs. Consequently, improving narrowly focused and expansive marketing strategies is essential.
2. Personalizing Digital Experiences
AI powers the optimization process, personalizing digital experiences to become more cost-effective and responsive to real-time user needs. Businesses can harness AI’s capabilities to make data-driven decisions by analyzing extensive volumes of data on user behavior and interactions. This results in enhancement in website performance coupled with increased user engagement. This underlines the critical role that AI-driven optimizations play in generating targeted marketing campaigns as well as personalized content creation, boosting conversion rates significantly.
3. Enhancing Content Creation and Optimization
Artificial intelligence optimization tools leverage user-search intent and preferences to automate content creation and optimization. Consequently, they offer insights into effective strategies for specific audiences. This enables them to produce content that resonates with their target audience. Furthermore, by deploying AI-driven tools that harness Natural Language Processing (NLP) models, these AI optimizations can enhance their outputs, ensuring engagement while making it error-free and tailoring it toward user needs.
4. Predicting Customer Behaviour
Machine learning algorithms, powered by AI optimization, predict customer behaviour. This enables marketers to anticipate customer needs and discern their preferences. The predictive capability of these tools proves particularly effective in e-commerce because personalized recommendations based on browsing and purchase history can amplify sales significantly.
5. Improving Customer Satisfaction
AI optimization intensifies customer satisfaction by delivering personalized experiences at scale. Decreasing response times and providing tailored product recommendations are among the many ways AI-powered virtual assistants and chatbots enhance the overall customer experience.Â
6. Saving Costs and Optimizing Resources
Through its capacity to automate repetitive tasks, AI optimization not only saves costs but also catapults productivity. Free of repetitive tasks, marketers can focus on more complex activities. This strategic reallocation of resources yields dual benefits for businesses: on the one hand, it sharpens marketing efforts, making them more precise and potent. On the other hand, it significantly enhances conversion rates and revenue. Moreover, by reducing reliance on human labor, AI integration notches up operational efficiency. This is borne out by an IBM survey of business organizations. It was found that 63% of people use AI to reduce manual or repetitive tasks.Â
ALSO READ: Top 20 Natural Language Learning Projects for Beginners to Pros
What are the Latest Trends and Advancements in AI Optimization Techniques?
There are constantly evolving trends and advancements in artificial intelligence optimization, significantly enhancing AI performance and efficiency. These developments reshape machine learning algorithm capabilities and refine AI model tuning processes. Some of these trends are presented below:
1. Enhanced Machine Learning Algorithms
Machine learning algorithm advancements have indeed focused on enhancing efficiency and accuracy, with techniques like deep learning, reinforcement learning, and transfer learning taking the lead. Such methods empower these algorithms to learn more effectively from extensive data volumes. Consequently, it enhances AI performance and enables them to solve complex problems with enhanced precision and reduced time.
2. AI Model Tuning With Automated Machine Learning (AutoML)
With the emergence of Automated Machine Learning (AutoML), significant improvements in AI model tuning have become evident. AutoML streamlines the selection process for optimal algorithms and hyperparameters tailored to specific tasks. This automation not only amplifies efficiency within AI applications but also broadens accessibility to non-experts who seek artificial intelligence optimization. Consequently, businesses are rendered able to deploy optimized AI models rapidly, which in turn accelerates their operations and enhances their decision-making processes.
3. Quantum Computing Integration
Quantum computing’s integration into artificial intelligence optimization signals a groundbreaking trend. With its potential to process information at unprecedented speeds, it accelerates and reshapes the optimization of AI models, particularly in areas demanding analysis of large data sets like drug discovery and financial modeling.
4. Federated Learning for Privacy-Preserving AI
An emerging trend, federated learning facilitates AI model training across multiple decentralized devices or servers while maintaining localized data. This strategy bolsters privacy and security; it directly confronts escalating concerns over data protection in AI applications. Furthermore, federated learning significantly boosts efficiency within artificial intelligence by diminishing the necessity for data centralization and empowering scalable AI solutions.
5. Energy-Efficient AI Models
The escalating concern for the environmental impact of computing has spurred a crucial trend: the development of energy-efficient AI models. With an aim to diminish the carbon footprint resulting from AI system training and deployment, researchers pivot toward crafting algorithms that demand lesser computational power; this not only fosters sustainability but also enhances accessibility by attenuating computation costs associated with AI.
ALSO READ: How to be Friends With AI. Yes, it’s Possible!
Want to learn more about artificial intelligence optimization? Consider joining Emeritus’ tailor-made artificial intelligence courses and machine learning courses, and boost your business.
Write to us at content@emeritus.org