Trend Alert: Physical AI is the Next Big Thing in AI

Physical AI (PAI) is essentially applying AI methods to address challenges that require direct engagement with the physical environment, such as seeing the world through sensors or manipulating the world using actuators. Physical AI is an emerging interdisciplinary field that primarily encompasses the foundational principles of the five sciences: chemistry, materials science, mechanical engineering, computer science, and biology. In fact, the lack of PAI skills has been the main obstacle in conducting successful research on developing realistic robots.
PAI focuses on addressing practical challenges that necessitate the capacity to observe and gather data in potentially expansive settings. It then involves processing and consolidating diverse data into formats suitable for automated reasoning. An example is to allow robots to make decisions or to assist humans in their everyday choices. Let’s understand the concept of Physical AI in greater detail.
Characteristics of Physical AI
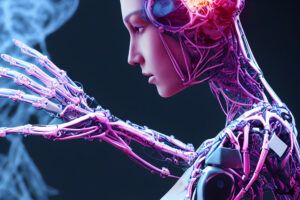
There are certain inherent characteristics of Physical AI. First is the ambiguity in the collected information, its incompleteness, and the uncertainty regarding the impact of actions on physical systems that coexist with humans in any given environment. Physical AI systems are, in fact, characterized by their direct engagement with the physical environment. This is primarily what sets them apart from other types of AI.
Let’s look at some examples. Financial recommendation systems involve AI acting as an intermediary between humans and a database. Then there are chatbots, which interact with humans through the Internet. AI chess players rely on humans to move the chess pieces and provide updates on the state of the chessboard to the AI algorithm.
Physical AI and Robotics
Current robots have several functions and different levels of “intelligence”. Traditional industrial robots perform monotonous activities on automated shop floors, for instance. Welding, assembling, and machining involve little sensitive interaction with the environment or humans. Likewise, service robots that interact with humans heavily depend on their sensors to perform navigation, object manipulation, and human-recognition tasks.
However, due to the intricate nature of their environment, the outcomes of their actions are only sometimes predictable. These robots exemplify systems that necessitate intelligence to navigate an unpredictable environment. They employ that intelligence to analyze data from physical sensors and physically interact with the world.
What is Unique About Physical AI?
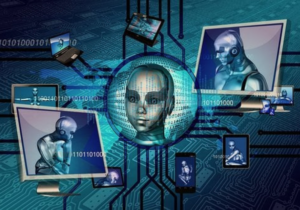
PAI systems extend beyond the realm of robotics. An option to enhance pollution detection capacities in cities is to deploy networks of cost-effective mobile micro-sensors that can be mounted in municipal electric automobiles. Their data can be utilized to estimate and categorize pollution levels directly from sensor data and input them into mathematical models.
For example, the vehicles carrying the sensors can be deliberately guided along routes that offer more data, utilizing appropriate decision-making algorithms. AI techniques can enhance pollution models’ accuracy by incorporating novel sensing and comprehension methods. City cameras can quantify mobile polluting sources such as autos and target crowds.
Why Physical AI is Important
The PAI methodology integrates considerations of materials, design, and manufacturing. Robots developed using PAI can autonomously perform tasks and maintain stability in unstructured environments by leveraging their bodies and brains’ physical and computational features. Like biological organisms, PAI robotics may replace or complement digital AI by interfacing with a “brain”. Some small robots may not have a central brain. Instead, they would rely on body computation.
Creating PAI is not without its challenges. It requires the integration of various disciplines, including materials science, mechanical engineering, computer science, chemistry, and biology. This paradigm shift from traditional robotics to PAI demands a blend of skills from these fields. Indeed, the diverse nature of skills required in each discipline highlights the venture’s complexity. However, acquiring expertise in such a broad range of skills is challenging. This is why more systematic educational approaches are needed to develop them.
Write to us at content@emeritus.org
NOTE: The views expressed in this article are those of the author and not of Emeritus.