Top 4 Common Mistakes in Data Analysis & Best Practices for A/B Testing
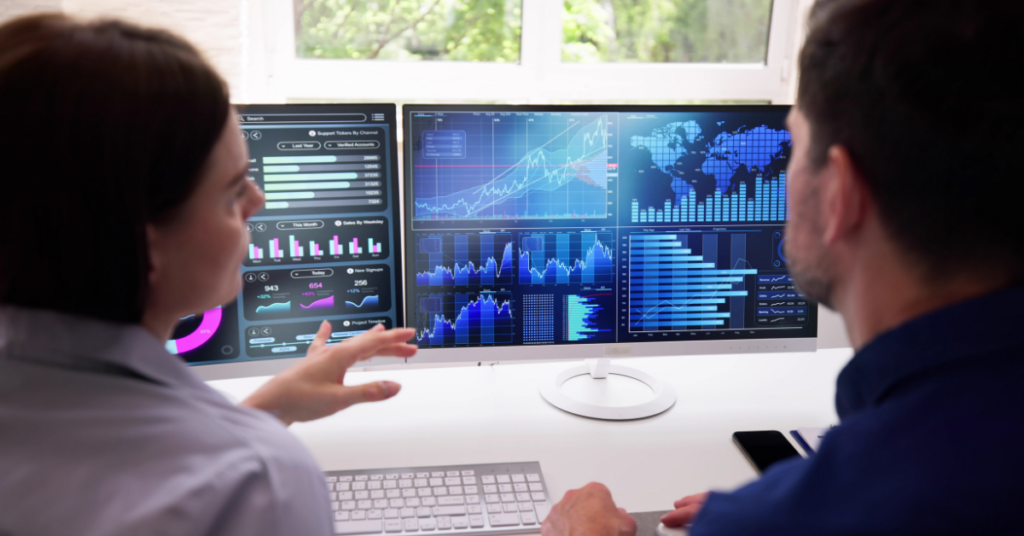
It is a given that common mistakes in data analysis often stem from overlooked details and assumptions. These errors can significantly skew results, leading to misguided business strategies. Therefore, recognizing and addressing these mistakes is crucial for accurate and reliable data interpretation. Moreover, this awareness is key to harnessing the full potential of data-driven decision-making.
And that brings us to A/B testing—also known as split testing—which compares two versions of a webpage or app against each other to determine which one performs better. Why A/B testing is important? To begin with, this approach is pivotal in data-driven decision-making.Here, decisions are based on data analysis and interpretation rather than intuition or observation alone. This method enhances the accuracy of decisions. It also allows for a nuanced understanding of user preferences and behaviors, helping you avoid common mistakes in data analysis.
 Effective data utilization can significantly impact business performance, leading to more informed and effective decisions. It plays a crucial role in enhancing the user experience as data-driven insights help in understanding and meeting customer needs more effectively. By leveraging data wisely, businesses can optimize their strategies, tailor their services, and achieve a competitive edge in their respective markets.
In this blog, we will discuss:
- The Basics of A/B Testing
- Common Data and A/B Testing Mistakes
- Best Practices for Effective A/B Testing
1. The Basics of A/B Testing
A. Explanation of A/B Testing
1. Split Testing
Before we delve into how to perform A/B testing, it’s important to understand that split testing is a core component of A/B testing. One of the common mistakes in data analysis involves dividing your audience to test variations of a product to determine which version yields the best results. Consequently, if you ask why A/B testing is important, it effectively isolates the impact of specific variables on user behavior, thus allowing for more targeted and effective modifications.
2. Randomized Control Trials
Randomized control trials are a scientific approach that needs to be understood to know how to perform A/B testing. It randomly assigns participants to a control group or a treatment group to measure the effect of a variable. Furthermore, it’s a cornerstone of rigorous scientific research and lends credibility and reliability to the results of A/B tests. To sum up, it lowers the possibility of common mistakes in data analysis.
ALSO READ: From Data to Excellence: The Top 10 Skills Every Data Scientist Needs
B. Key Components
1. Control Group
The control group in A/B testing serves as a benchmark. While it receives no experimental changes, it does allow for comparisons against the treatment group. Understanding the impact of the changes being tested through this comparison is crucial for avoiding common mistakes in data analysis.
2. Treatment Group
In A/B testing, the treatment group undergoes exposure to the experimental changes. Additionally, researchers compare this group’s performance with that of the control group to gauge the effectiveness of these changes. The insights gained here are invaluable for iterative development and optimization.
3. Hypothesis Formation
Hypothesis formation is a critical step in A/B testing. It requires creating a testable statement based on assumptions. The testing process then validates or invalidates the statement accordingly. Therefore, a well-formed hypothesis guides the entire testing process, ensuring that the test remains focused and relevant.
2. Common Mistakes in Data Analysis
A. Misinterpreting Data
1. The Danger of Relying on Surface-Level Insights
One of the common mistakes in data analysis is relying on surface-level insights without delving deeper into the data. This can, evidently, lead to misguided decisions. Moreover, this superficial approach can also overlook underlying trends and anomalies that are critical for informed decision-making.
2. The Importance of Statistical Significance
 It is important to understand the concept of statistical significance in data analysis. This helps in determining whether the results of the test represent actual differences between groups. Ignoring this can result in erroneous conclusions being drawn from random variations in data.
B. Inadequate Sample Size
1. Impact on Reliability
Inadequate sample size can significantly affect the reliability of A/B testing results, leading to inaccurate conclusions. Thus, a sample size that is too small may not accurately represent the larger population. This, in turn, leads to skewed results.
2. Calculating the Right Sample Size
It’s essential to calculate the right sample size for your A/B test to ensure the results are statistically valid. Again, this involves considering the expected effect size, the variability in the data, and the desired level of confidence in the results.
ALSO READ: Building a Data-Driven Culture: Top 10 Skills for Data Literacy
C. Biased Sampling
1. Understanding Different Types of Bias
Understanding the different types of bias in sampling is another crucial step in this process. As a matter of fact, these biases can skew the results of an A/B test, leading to incorrect conclusions. Therefore, recognizing and addressing these biases is essential for the integrity of the test.
2. Strategies to Mitigate Bias
Implementing strategies to mitigate bias is essential for obtaining accurate and reliable results from A/B testing. This might involve random sampling techniques or ensuring a representative sample of the population is included in the test.
D. Overlooking External Factors
1. Identifying External Influences
Identifying external influences that can affect A/B testing results is a critical step in ensuring accurate data analysis. Additionally, factors such as seasonal variations, market trends, or external events can significantly impact the results.
2. Adjusting for External Variables in Analysis
Moreover, making adjustments for external variables in the analysis is necessary. It helps isolate the effect of the variable being tested in A/B testing. This ensures that the results are attributable to the changes made in the test rather than external factors.
ALSO READ: 10 Top In-Demand Jobs in India to Future-Proof Your Career in 2024
3. Best Practices for Effective A/B Testing
A. Clear Hypothesis Formulation
1. Importance of a Clear Hypothesis
Formulating a clear hypothesis becomes paramount for effective A/B testing. It provides a focused direction for the test and helps in measuring specific outcomes. Moreover, a clear hypothesis also facilitates clearer communication and understanding among team members and stakeholders.
2. Structuring Hypotheses for Effective Testing
Structuring hypotheses effectively is key to conducting successful A/B tests. One of the best ways to avoid common mistakes in data analysis is to have a well-structured hypothesis. The hypothesis should be testable, specific, and measurable. It must be grounded in research and existing data to ensure its relevance and potential impact.
B. Randomization and Control
1. Ensuring Unbiased Testing Conditions
Ensuring unbiased testing conditions through randomization is essential in A/B testing. This approach helps eliminate selection bias. It ensures that the results are reflective of true performance rather than artifacts of the sample selection process.
2. Proper Control Group Setup
Another vital element is setting up a proper control group for comparing test results effectively in A/B testing. The control group must be comparable to the treatment group in all respects except for the variable being tested.
C. Statistical Significance
1. Interpreting P-Values and Confidence Intervals
Interpreting P-values and confidence intervals correctly is crucial in A/B testing. These statistical measures help in understanding the significance of the test results. Moreover, they provide a quantifiable measure of how likely it is that the observed results are due to chance.
2. Setting Significance Thresholds
Setting appropriate significance thresholds is important in determining the success or failure of an A/B test. These thresholds help in making informed decisions about whether to implement the changes tested.
ALSO READ: How an Advanced Certificate Program in Data Science Will Help You Break Into the Industry
D. Continuous Monitoring
1. The Importance of Ongoing Analysis
Continuous monitoring and ongoing analysis are essential in A/B testing. This approach helps in identifying trends and making timely adjustments. Additionally, it ensures that the test remains relevant and accurate throughout its duration.
2. Identifying Trends and Anomalies
Identifying trends and anomalies during A/B testing is crucial for understanding the effectiveness of the changes being tested. This ongoing analysis allows for quick responses to unexpected results or emerging patterns.
ALSO READ: Top 10 High-Income Skills to Learn in 2024 to Make a Six-Figure Salary
Learn Data Analysis With Emeritus
In conclusion, understanding and avoiding the above-mentioned common mistakes in data analysis is crucial for successful A/B testing. By following best practices and continuously learning, you can significantly improve your data analysis skills. For those interested in further enhancing their expertise, consider exploring these data science courses by Emeritus. They can deepen your understanding and application of these concepts.
Write to us at content@emeritus.org