Understanding the Ethics of Data Analytics: A Comprehensive Guide
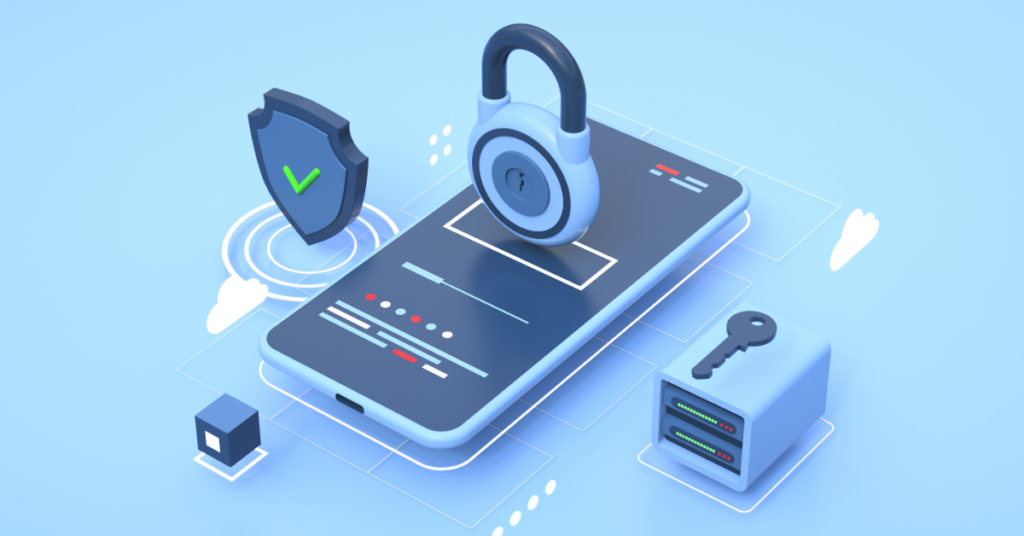
The ethics of data analytics is not just a set of theoretical concepts but is deeply rooted in practical applications that impact everyday decision-making. As we increasingly rely on data to guide business strategies, policymaking, and even personal choices, the ethical implications of how this data is collected, processed, and interpreted become more significant. This blog navigates the nuances of data privacy laws, such as the General Data Protection Regulation (GDPR), and their impact on analytics practices. It will also examine how ethical considerations are vital in maintaining trust and integrity in data analytics.
In this blog, we will discuss:
- Data Regulations
- Ethics of Data Analytics
- Algorithmic Bias
- Future Trends in the Ethics of Data Analytics
Data Regulations
A. Overview of Data Protection Laws
-
GDPR (General Data Protection Regulation)
The General Data Protection Regulation (GDPR) has set a new benchmark for data privacy, particularly for EU citizens. This regulation is emblematic of the ethics of data analytics, highlighting rigorous data handling and processing practices. It mandates transparency in data processing, has stringent consent requirements, and gives individuals significant control over their data. Furthermore, it imposes hefty penalties for non-compliance, ensuring that organizations prioritize data protection.
-
CCPA (California Consumer Privacy Act)
The California Consumer Privacy Act (CCPA) emerged as a pioneering legislation in the US. This act is instrumental in shaping the future of privacy laws nationwide. For instance, it introduces rights such as the ability to know about and opt out of the sale of personal information, access to data, and the right to request deletion of personal information. Thus, the CCPA is not only a legislative framework but also a reflection of the growing demand for privacy rights in the digital era.
-
Other Global and Regional Regulations
The recognition of data privacy as a fundamental right is gaining momentum globally. For instance, Brazil’s LGPD (Brazilian Data Protection Law) echoes the GDPR’s principles, focusing on transparency, accountability, and the need for clear consent. Similarly, India’s Digital Personal Data Protection Act 2023 seeks to empower individuals with rights similar to the GDPR, indicating a global trend toward robust data privacy norms. In essence, these laws signify a paradigm shift in how personal data is perceived and managed worldwide, underscoring the universal importance of data privacy in the context of ethics of data analytics.Â
ALSO READ: What is Big Data? Let’s Analyze its Rise and Implications
B. Compliance Challenges for Analytics
-
Consent Management
Consent management is a critical aspect of the compliance framework, ensuring that users’ preferences regarding data usage are not only respected but also strictly adhered to. This process involves obtaining explicit and informed consent from users before collecting or processing their data. Moreover, it provides clear and accessible information about how the data will be used, empowering users to make informed decisions. Additionally, organizations must ensure that the process for withdrawing consent is as straightforward as the process for giving it, upholding the principles of fairness and respect for user autonomy.
-
Data Minimization
Data minimization advocates for the collection and processing of only the data that is essential for the specified purpose. In essence, this principle plays a pivotal role in safeguarding user privacy by limiting the scope of data exposure and reducing the risk of data breaches. Additionally, it encourages organizations to critically evaluate their data collection practices, ensuring that every piece of data collected serves a legitimate and necessary purpose.
ALSO READ: A Deep Dive Into Data Lakes: 7 Best Practices for Data Management
-
Right to Access and Data Portability
The rights to access and data portability are fundamental components of modern data privacy laws. The right to access allows individuals to request and receive a copy of their data held by an organization, promoting transparency and accountability. On the other hand, the right to data portability enables individuals to transfer their data from one service provider to another, fostering competition and allowing users to choose services that fit their privacy expectations.Â
C. Implications of Noncompliance
-
Legal Consequences
Legal consequences, often severe, can lead to substantial fines. These fines serve as a deterrent, emphasizing the importance of regulatory adherence. Moreover, legal actions against violators can result in lengthy litigations. Such legal entanglements not only drain resources but also divert attention from core business activities.
-
Reputational Risks
Reputational risks can irreparably damage a company’s public image. Consequently, this damage often leads to a significant loss of consumer trust. The loss of trust can, in turn, result in decreased customer loyalty. Furthermore, it can hinder the acquisition of new clients, impacting long-term business growth and stability.
Ethics of Data Analytics
A. Defining the Term
Ethics of data analytics revolve around a set of moral principles that are essential in guiding the use and analysis of data. These principles ensure that data handling is compliant with legal standards and aligns with broader moral and ethical values. For instance, principles like data privacy, accuracy, and transparency are not just regulatory requirements but also ethical imperatives. They ensure that data is used in a way that respects individual rights and societal norms. Moreover, ethical data analytics involves considering the potential impacts of data decisions on various stakeholders, including those who provide the data and those affected by data-driven decisions. This approach is vital in maintaining a balance between leveraging data for its utility and respecting ethical considerations such as privacy, fairness, and non-discrimination.Â
ALSO READ: What is Data Mining and How to Make a Good Career in it
B. Responsible Data Use
-
Privacy Concerns
Privacy concerns, paramount in data analytics, necessitate stringent measures. These measures protect user data from misuse. Additionally, they align with regulations like the general data protection regulation, ensuring compliance and ethical handling of data.
-
Informed Consent
Informed consent is a critical aspect of ethical data use. It ensures users are fully aware of how their data is utilized. Moreover, it empowers users to make informed decisions about their data, enhancing the ethical framework within which data analytics operates.
C. Fairness and Transparency
-
Avoiding Discrimination
Avoiding discrimination is not just a legal requirement but a moral obligation. It ensures that analytics practices do not perpetuate or amplify biases. Furthermore, it fosters an environment of equality in data-driven decision-making.
-
Openness in Algorithms
Openness in algorithms is crucial for fostering trust among users. It involves transparently disclosing how algorithms process data. This transparency allows users to understand and trust the decision-making processes, reinforcing the ethical foundations of data analytics.
ALSO READ: 9 Data Analytics Books For Beginners
D. Ethical Decision-Making Frameworks
-
Utilitarianism
Utilitarianism focuses on achieving the greatest good for the greatest number. In data analytics, this approach evaluates the overall impact of decisions on society. In essence, it involves analyzing the benefits and drawbacks of data usage, aiming to maximize positive outcomes while minimizing harm. For instance, when utilizing user data for research, a utilitarian approach would weigh the societal benefits of the research against the potential privacy risks to individuals. This framework encourages decisions that contribute to the overall well-being and progress of society.
-
Deontology
Deontology, another crucial ethical perspective, adheres strictly to rules and duties. In the context of data analytics, it emphasizes adherence to ethical obligations and principles, regardless of the outcomes. This approach prioritizes the ethical process over the end result, ensuring that data is handled in an inherently right and just manner. For example, deontology in data analytics would insist on transparent data practices and respect for user consent, upholding ethical standards even if it means foregoing certain analytical insights.
-
Virtue Ethics
Virtue ethics, focusing on moral character, emphasizes the virtues of decision-makers in data analytics. In the context of ethics of data analytics, this framework advocates for qualities like honesty, integrity, and fairness in the handling and analysis of data. It encourages professionals to cultivate good character traits, leading to ethical decisions naturally. In practice, virtue ethics would guide a data analyst to respect user privacy and data integrity, not merely as a compliance requirement but as a reflection of their moral character.
ALSO READ: 4 Best Practices for Data Visualization in Matplotlib
Algorithmic Bias
A. Understanding Algorithmic Bias
Algorithmic bias occurs when algorithms yield skewed results, often leading to unfair outcomes. This bias can arise unintentionally due to underlying issues in the data or the algorithm’s design. In the context of the ethics of data analytics, understanding and addressing algorithmic bias is crucial, as it aligns with the regulation’s emphasis on fairness and transparency in data processing.
B. Types of Bias in Analytics
-
Sampling Bias
Sampling bias emerges from unrepresentative data samples, skewing analysis results. This bias can lead to misleading conclusions, as the data does not accurately reflect the broader population. Hence, it is a direct challenge to the ethics of data analytics, as it can lead to decisions that are not truly representative or fair.
-
Selection Bias
Selection bias occurs during the data selection phase, affecting the accuracy of outcomes. This bias happens when certain data is chosen over others, potentially leading to partial or skewed results. In the context of what is general data protection regulation, addressing selection bias is vital to ensure that data processing is fair and unbiased.
-
Measurement Bias
Measurement bias, related to data collection methods, can distort analysis findings. It arises when the data collection tools or techniques are flawed, leading to inaccurate or biased data. In essence, this type of bias challenges the ethics of data analytics by compromising the quality and reliability of the data.
C. Impact of Bias in Decision-Making
-
Social and Economic Consequences
These consequences can exacerbate social and economic inequalities, affecting individuals and communities. Biased decision-making can lead to unfair practices and policies, disproportionately impacting certain groups. Hence, this issue is particularly relevant in the context of data protection.
-
Reinforcement of Stereotypes
Bias in analytics can reinforce harmful stereotypes, perpetuating existing societal biases. This reinforcement affects the stereotyped groups and skews the overall understanding and interpretation of data.Â
D. Mitigating Algorithmic Bias
-
Fairness-Aware Machine Learning
This approach incorporates fairness considerations into algorithm design to reduce bias in machine learning models. It is a proactive step toward aligning with what is general data protection regulation, ensuring that algorithms treat all data fairly and without prejudice.
ALSO READ: How is Python Crafting Brilliance in Model-Based Clustering Algorithms?
-
Regular Audits and Assessments
Conducting regular audits and assessments helps maintain ongoing fairness in analytics. These practices are crucial for identifying and addressing biases, ensuring that the analytics processes remain aligned with the ethics of data analytics. Regular audits also help organizations comply with regulations like the general data protection regulation, fostering a culture of accountability and continuous improvement.
Future Trends in Ethics of Data AnalyticsÂ
A. Evolving Data Regulations
-
Potential New Legislation
Emerging legislation may address new challenges in data privacy and security. For instance, laws might focus on using emerging technologies like facial recognition and deep learning ethically. These laws will likely extend the principles of data protection regulation to newer realms of technology, ensuring that advancements in data analytics remain aligned with privacy and ethical standards.
-
Global Harmonization Efforts
Efforts toward global harmonization, such as the proposed ePrivacy Regulation in the EU, aim to standardize data protection laws across borders. This initiative seeks to create a cohesive framework, making it easier for organizations to comply with various international regulations and reinforcing the principles of what is general data protection regulation on a global scale. The Digital Personal Data Protection Act 2023 in India also aligns with these global efforts, aiming to establish a robust data protection ecosystem that resonates with international standards.
B. Advancements in Ethical AI
-
AI Ethics Research
Research in AI ethics is delving into areas like algorithmic fairness and transparency. Institutions like the AI Now Institute are exploring how AI decisions can impact human rights, and tools like Google’s AI Ethics Checklist are being developed to guide ethical AI development.
ALSO READ: Difference Between Data Science, Artificial Intelligence and Machine Learning
-
Integration of Ethical Considerations in AI Development
Integrating ethics into AI development involves principles like explainability and accountability. Frameworks such as IEEE’s (Institute of Electrical and Electronics Engineers) Ethically Aligned Design are being adopted to ensure AI systems are developed with ethical considerations at their core, reflecting the ethics of data analytics.
C. Innovations in Bias Mitigation
-
Emerging Technologies
Emerging technologies are at the forefront of combating biases in machine learning models. Tools like IBM’s Fairness 360 Toolkit represent a significant stride in this direction. They are designed to identify and correct biases in both data and algorithms. Other technologies, such as Google’s What-If Tool, provide an interactive interface for practitioners to visualize and assess the impact of bias in their models. In essence, these tools are instrumental in aligning analytics practices with the ethics of data analytics, ensuring decisions made by AI are fair and equitable.
-
Industry Best Practices
The establishment of industry best practices is another critical step towards mitigating bias. Regular ethical audits, for instance, involve scrutinizing algorithms and data sets to identify potential biases. This practice ensures ongoing vigilance and correction of biases, aligning with the general data protection regulation principles. Additionally, it emphasizes using diverse datasets, which involves collecting data from varied and inclusive sources to prevent the skewing of machine learning outcomes. Such practices not only enhance the fairness of algorithms but also ensure that the insights derived from data analytics are representative of diverse populations, adhering to the ethics of data analytics.
ALSO READ: 5 Ways Big Data Analytics is Reshaping the Business Landscape in India
The ethics of data analytics are fundamental in shaping a data-driven world that is not only efficient and innovative but also fair and respectful of individual rights and societal values. For those keen on diving deeper into this fascinating field and navigating its complexities, exploring Emeritus’ tailor-made data science courses can provide valuable insights and skills.
Write to us at content@emeritus.orgÂ