Tidy Data Demystified: Why It Matters in Data Science
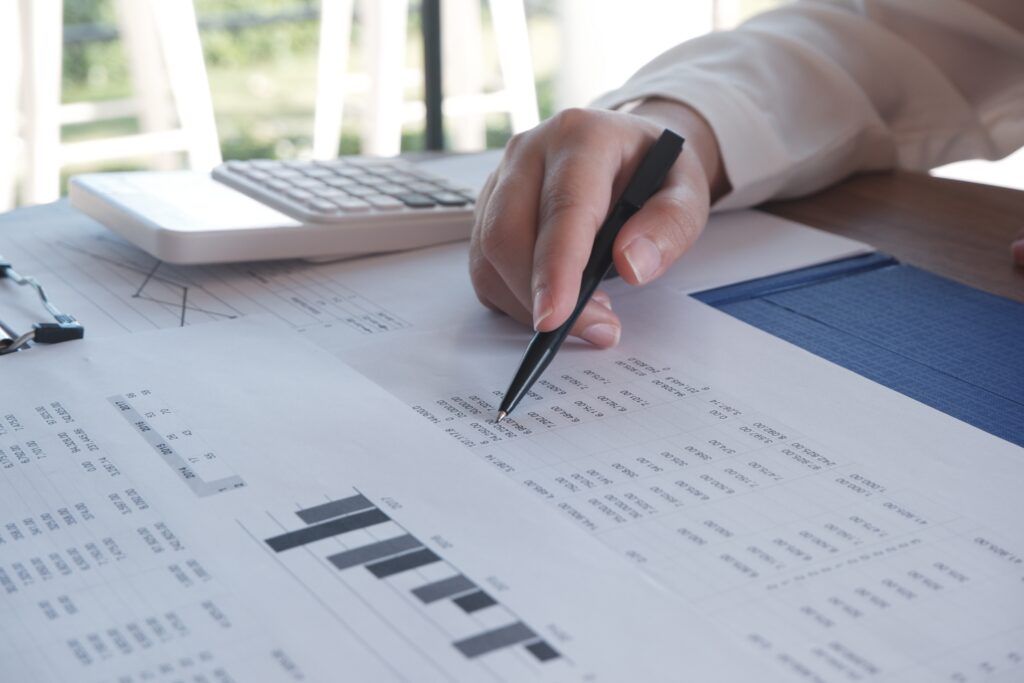
A common saying is that data scientists spend 80% of their time cleaning and preparing data and only 20% on actual analysis. This means that in a typical data science project, most of the time is dedicated to data cleaning. Surprisingly, there aren’t many tools or data science approaches on how to do this effectively. However, one notable research contribution comes from Hadley Wickham, a New Zealand-based statistician, who introduced the concept of tidy data—the process of organizing data sets in a way that simplifies data analysis. With tidy data, data scientists can save the time spent on cleaning and preparing data and utilize the time for data analysis.
In this article, we explore what tidy data is, why it is important, and how it benefits data analysis.
In this blog, you’ll learn:
- What is Tidy Data, and Why is it Essential?
- How Does Tidy Data Benefit Businesses and Industries?
- What are the Challenges Involved in Achieving Tidy Data?
- How Can Individuals and Organizations Adopt Tidy Data Principles for Better Data Handling?
- Start Your Data Science Journey With Emeritus
What is Tidy Data, and Why is It Essential?
There are different ways of organizing data. Some of these ways make for easy data analysis, while others lead to a lot of confusion. This is where tidy data comes in. A concept introduced in Hadley Wickham in 2014 it refers to the process of providing clean and organized data. Furthermore, tidy data is often used in data analysis and manipulation, especially in the R programming language. It helps to streamline data cleaning, making it easier for analysts to perform tasks such as visualization and modeling.
This is an essential aspect related to analyzing data because poor data quality hinders the strategic decision-making capabilities of an organization. Moreover, it can cost businesses an average of $12.9 million in losses annually. Besides its immediate revenue impact, the poor quality of data over the long term adds complexity to data ecosystems and hampers effective decision-making. This is why tidy data is crucial for streamlining processes and even driving innovation.
ALSO READ: Implications of Data Visualization in Data Analytics
How Does Tidy Data Benefit Businesses and Industries?
1. Simplified Data Analysis and Insights
Tidy data simplifies data analysis by providing a standardized structure where variables are represented as columns. This allows data analysts to perform statistical tests, generate meaningful visualizations, and uncover actionable insights more efficiently. Moreover, it reduces the time spent on data preparation, enabling quicker decision-making and a competitive edge in dynamic markets.
2. Improved Data Visualization
Another significant benefit is that the organized format of Tidy data is especially conducive to data visualization. Data scientists and business analysts can easily create visual representations of data, such as bar charts, scatter plots, and graphs, where each variable corresponds to a visual element. This enhances the communication of complex information to both technical and non-technical stakeholders, facilitating easy information handling and informed decision-making.
3. Easier Data Integration
Many industries rely on data from various sources, including databases, web services, and Internet of Things (IoT) devices. Tidy data simplifies the integration of disparate data sets, thus reducing the time and effort needed to consolidate information. It consequently ensures a smooth flow of data between systems and departments, enabling organizations to harness the full potential of big data analytics.
4. More Accurate Use of Machine Learning and Artificial Intelligence (AI)
Machine learning algorithms require structured input data for training and prediction. In this scenario, tidy data’s consistent format streamlines data preprocessing, allowing organizations to build accurate models and leverage artificial intelligence for predictive analytics and automation.
5. Regulatory Compliance
Finally, compliance with data standards is paramount in highly regulated sectors such as health care and finance. Tidy data, therefore, simplifies regulatory compliance by providing a clear structure for data storage and retrieval. This ensures that businesses can meet audit and reporting requirements efficiently, reducing the risk of penalties and reputational damage.
ALSO WATCH: Mid-Career Transition to Data Science by Dr Chirnajiv Roy, Head of Data Science & Analytics
What are the Challenges Involved in Achieving Tidy Data?
Achieving tidy data comes with several challenges that can impact data quality, analysis, and decision-making. Here are some of them:
-
Data Integration and Collection
First and foremost, it is challenging to gather data from diverse sources because of varying formats and inconsistencies.
-
Handling Messy Data
Then, there is the fact that it’s also hard to manage data with multiple observations in single cells, nonstandard delimiters, or inconsistent column names.
-
Data Governance
Establishing clear guidelines for data entry, naming conventions, and updates to maintain tidiness over time is difficult.
-
Data Volume
Another challenge is handling large data sets efficiently because this necessitates substantial computational resources.
-
Data Quality Assessment
Regularly assessing data quality, detecting anomalies, and addressing them promptly is another challenge to achieving tidy data.
-
Missing Data
Next is the challenge of developing strategies for handling missing values, such as imputation or exclusion.
-
Duplicated Data
Identifying and removing duplicate entries to prevent distorted analysis results.
-
Scalability
Lastly, adapting tidy data practices for big data with specialized tools and infrastructure.
ALSO READ: 10 Best Data Visualization Tools in 2023
How Can Individuals and Organizations Adopt Tidy Data Principles for Better Data Handling?
1. Education and Training
Individuals and teams should engage in comprehensive training programs to gain proficiency in data management and tidy data principles. Enrolling in online courses, attending workshops, and obtaining relevant certifications can empower professionals with the knowledge and skills needed to effectively clean up data.
2. Data Governance Policies
Organizations must develop and implement robust data governance policies. These policies should emphasize the importance of data quality, standardized data formats, and adherence to tidy data practices. Ensuring that these policies are clearly communicated and understood across the organization is crucial.
3. Data Cleaning Tools
Employing specialized data cleaning and transformation tools can significantly streamline data preparation. Tools such as OpenRefine, Trifacta, or TIBCO Clarity offer automation capabilities, making data cleaning processes more efficient and less error-prone.
4. Regular Auditing and Monitoring
Implementing regular data audits and monitoring mechanisms is essential. These procedures further help in identifying any deviations from tidy data principles. Moreover, they also trigger corrective actions promptly, ensuring data quality is maintained.
5. Collaboration and Communication
Alco encourages collaboration between data professionals, domain experts, and data consumers. Doing so is vital because effective communication will ensure that data is structured in a way that aligns with analytical needs and business objectives.
6. Machine Learning and AI Integration
Finally, consider how tidy data principles can be seamlessly integrated into machine learning and AI pipelines. Clean and structured data is the foundation for training and deploying AI models, enhancing model performance and interpretability.
ALSO WATCH: Thinking About Career Transition? Let’s Talk! by Ruchika Rocque, HR, Microsoft
Start Your Data Science Journey With Emeritus
Planning to start your data science journey? Explore data science courses from the world’s best universities on Emeritus. These courses are designed for professionals from any domain looking to advance their careers in data science. Moreover, these courses will equip learners with skills that will give them insight into modern data science practices.
Write to us at content@emeritus.org